subscribe to our mailing list:
|
SECTIONS
|
 |
 |
 |
Not a Free Lunch But a Box of Chocolates
A critique of William Dembski's book No Free Lunch
Version 1.0 [Last Modified: April 23, 2002]
Permission is given to copy and print
this page for non-profit personal or educational use.
Contents
Summary
1. Introduction
2. Design and Nature
3. The Chance-Elimination Method
4. Applying the Method to Nature
5. Evolutionary Algorithms
6. The Uniform-Probability Method
7. The Positive Case For Design
8. Dembski and Peer
Review 9.
Conclusion Acknowledgements Appendix.
Dembski's Statistical Method Examined Notes
Summary
Life is like a box of chocolates. You never know what you're
gonna get. Forrest Gump
The aim of Dr William
Dembski's book No Free Lunch is to demonstrate that design (the action of
a conscious agent) was involved in the process of biological evolution. The
following critique shows that his arguments are deeply flawed and have little to
contribute to science or mathematics. To fully address Dembski's arguments has
required a lengthy and sometimes technical article, so this summary is provided
for the benefit of readers without the time to consider the arguments in
full.
Dembski has proposed a method of inference which, he claims, is a rigorous
formulation of how we ordinarily recognize design. If we can show that an
observed event or object has low probability of occurring under all the
non-design hypotheses (explanations) we can think of, Dembski tells us to infer
design. This method is purely eliminative--we are to infer design when we have
rejected all the other hypotheses we can think of--and is commonly known as an
argument from
ignorance, or god-of-the-gaps argument.
Because god-of-the-gaps arguments are almost universally recognized by
scientists and philosophers of science to be invalid as scientific inferences,
Dembski goes to great length to disguise the nature of his method. For example,
he inserts a middleman called specified
complexity: after rejecting all the non-design hypotheses we can think
of, he tells us to infer that the object in question exhibits specified
complexity, and then claims that specified complexity is a reliable indicator of
design.
The only biological object to which Dembski applies his method is the
flagellum of the bacterium E. coli. First, he attempts to show that the
flagellum could not have arisen by Darwinian evolution, appealing to a modified
version of Michael Behe's argument from irreducible
complexity. However Dembski's argument suffers from the same fundamental
flaw as Behe's: he fails to allow for changes in the function of a biological
system as it evolves.
Since Dembski's method is supposed to be based on probability and he has
promised readers of his earlier work a probability calculation, he proceeds to
calculate a probability for the origin of the flagellum. But this calculation is
based on the assumption that the flagellum arose suddenly, as an utterly random
combination of proteins. The calculation is elaborate but totally
irrelevant, since no evolutionary biologist proposes that complex biological
systems appeared in this way. In fact, this is the same straw man assumption
frequently made by Creationists in the past, and which has been likened to a
Boeing 747 being assembled by a tornado blowing through a junkyard.
This is all there is to Dembski's main argument. He then makes a secondary argument
in which he attempts to show that even if complex biological systems did evolve
by undirected evolution, they could have only done so if a designer had
fine-tuned the fitness function or inserted complex specified
information at the start of the process.
The argument from fine-tuning of fitness
functions appeals to a set of mathematical theorems called the "No Free Lunch"
theorems. Although these theorems are perfectly sound, they do not have the
implications which Dembski attributes to them. In fact they do not apply to
biological evolution at all. All that is left of Dembski's argument is then
the claim that life could only have evolved if the initial conditions of the
Universe and the Earth were finely tuned for that purpose. This is an old
argument, usually known as the argument from cosmological (and terrestrial)
fine-tuning. Dembski has added nothing new to it.
Complex specified
information (CSI) is a concept of Dembski's own invention which is quite
different from any form of information used by information theorists. Indeed,
Dembski himself has berated his critics in the past for confusing CSI with other
forms of information. This critique shows that CSI is equivocally defined and
fails to characterize complex structures in the way that Dembski claims it does.
On the basis of this flawed concept, he boldly proposes a new Law of Conservation of
Information, which is shown here to be utterly
baseless.
Dembski claims to have made major contributions to the fields of statistics,
information theory and thermodynamics. Yet his work has not been
accepted by any experts in those fields, and has not been published in any
relevant scholarly journals.
No Free Lunch consists of a collection of tired old antievolutionist
arguments: god-of-the-gaps, irreducible complexity, tornado in a junkyard, and
cosmological fine-tuning. Dembski attempts to give these old arguments a new
lease of life by concealing them behind veils of confusing terminology and
unnecessary mathematical notation. The standard of scholarship is abysmally low,
and the book is best regarded as pseudoscientific rhetoric aimed at an unwary
public which may mistake Dembski's mathematical mumbo jumbo for academic
erudition.
1. Introduction
In the theater of confusion, knowing the location of the
exits is what counts. Mason Cooley, U.S.
aphorist
William Dembski's book No Free Lunch: Why Specified Complexity Cannot be
Purchased without Intelligence1 is
the latest of his many books and articles on inferring design in biology, and
will probably play a central role in the promotion of Intelligent Design
pseudoscience2 over
the next few years. It is the most comprehensive exposition of his arguments to
date. The purpose of the current critique is to provide a thorough critical
examination of these arguments. Dembski himself has often complained that his
critics have not fully engaged his arguments. I believe that complaint is
unjustified, though I would agree that some earlier criticisms have been poorly
aimed. This critique should lay to rest any such complaints.
As in his previous work, Dembski defines his own terms poorly, gives new
meanings to existing terms (usually without warning), and employs many of these
terms equivocally. His assertions often appear to contradict one another. He
introduces a great deal of unnecessary mathematical notation. Thus, much of this
article will be taken up with the rather tedious chore of establishing just what
Dembski's arguments and claims really mean. I have tried very hard to find
charitable interpretations, but there are often none to be found. I have also
requested clarifications from Dembski himself, but none have been
forthcoming.
Some time ago, I posted a critique3 of
Dembski's earlier book, The Design Inference,4 to the
online Metaviews forum, to which he contributes, pointing out the fundamental
ambiguities in his arguments. His only response was to call me an "Internet
stalker" while refusing to address the issues I raised, on the grounds that "the
Internet is an unreliable forum for settling technical issues in statistics and
the philosophy of science".5 He
clearly read my critique, however, since he now acknowledges me as having
contributed to his work (p. xxiv). While some of the ambiguities I drew
attention to in that earlier critique have been resolved in his present volume,
others have remained and many new ones have been added.
Some readers may dislike the frankly contemptuous tone that I have adopted
towards Dembski's work. Critics of Intelligent Design pseudoscience are faced
with a dilemma. If they discuss it in polite, academic terms, the Intelligent
Design propagandists use this as evidence that their arguments are receiving
serious attention from scholars, suggesting this implies there must be some
merit in their arguments. If critics simply ignore Intelligent Design arguments,
the propagandists imply this is because critics cannot answer them. My solution
to this dilemma is to thoroughly refute the arguments, while making it clear
that I do so without according those arguments any respect at all.
This critique assumes a basic knowledge of mathematics, probability theory
and evolutionary theory on the part of the reader. In order to simplify some of
my arguments, I have relegated many details to endnotes, which can be reached by
numbered links. In some cases, assertions which are not substantiated in the
body of the text are supported by arguments in endnotes.
Citations consisting merely of page numbers refer to pages in No Free
Lunch.
Regrettably, some older browsers are unable to display a number of
mathematical symbols which are used in this article. Netscape 4 is one of
these.
2. Design and Nature
In spring, when woods are getting green, I'll try and tell
you what I mean. Lewis Carroll, Through the
Looking-Glass (Humpty Dumpty)
For a book which is all about inferring design, it is surprising to
discover that No Free Lunch does not clearly define the term.
Design is equated with intelligent agency, but that term is not
defined either. It is also described negatively, as the complement of necessity
(deterministic processes) and chance (stochastic processes). However,
deterministic and stochastic processes are themselves normally defined as
mutually exhaustive complements: those processes which do not involve any
uncertainty and those which do. So it is not clear what, if anything, remains
after the exclusion of those two categories. Dembski associates design
with the actions of animals, human beings and deities, but seems to deny the
label to the actions of computers, no matter how innovative their output may be.
What distinguishes an animal mind, say, from a computer? Obviously there are
many physical differences. But why should the actions of one be considered
design and not the other? The only explanation I can think of is that one is
conscious and the other, presumably, is not. I conclude that, when he infers
design, Dembski means that a conscious mind was involved.
It appears that Dembski considers consciousness to be a very special kind of
process, which cannot be attributed to physical laws. He tells us that
intelligent design is not a mechanistic explanation (pp. 330-331). Dembski would
certainly not be alone in this view, though it is not at all clear what it means
for a process to be non-mechanistic. It appears, however, that such a process is
outside the realm of cause and effect. This raises all sorts of difficult
philosophical questions, which I will not attempt to consider here. Even if we
accept that non-mechanistic processes exist, Dembski gives us no reason to think
that consciousness (or intelligent design) is the only possible type of
non-mechanistic process. Yet he seems to assume this to be the case.
Even with this interpretation, we still run into a problem. In his Caputo
example (p. 55), Dembski uses his design inference to distinguish between two
possible explanations both involving the actions of a conscious being: either
Caputo drew the ballots fairly or he cheated. Dembski considers only the second
of these alternatives to be design. But both explanations involve a conscious
agent. It could be said that, if Caputo drew fairly, he was merely mimicking the
action of a mechanistic device, so this doesn't count. But that would raise the
question of just what a mechanistic device is capable of doing. Is a
sophisticated computer not capable of cheating? Indeed, is there any action of a
human mind which cannot, in principle, be mimicked by a sufficiently
sophisticated computer? If not, how can we tell the difference between conscious
design and a computer mimicking design? Even if you doubt that in principle a
computer could mimic all the actions of a human mind, consider whether it could
mimic the actions of a rat, which Dembski also considers to be an intelligent
agent capable of design (pp. 29-30).
To escape this dilemma, Dembski invokes the concept of derived
intentionality: the output of a computer can "exhibit design", but the
design was performed by the creator of the computer and not by the computer
itself (pp. 223, 326). Whenever a phenomenon exhibits design, there must be a
designer (a conscious mind, in my interpretation) somewhere in the causal chain
of events leading to that phenomenon.
Dembski claims that contemporary science rejects design as a legitimate mode
of explanation (p. 3). But he himself gives examples of scientists making
inferences involving human agency, such as the inference by archaeologists that
certain stones are arrowheads made by early humans (p. 71), and he labels these
"design inferences". Is he claiming that such archaeologists are mavericks
operating outside the bounds of mainstream science? I don't think so. I think
that what Dembski really means to claim here is that contemporary science does
not allow explanations involving non-mechanistic processes, and he is projecting
his own belief that design is a non-mechanistic process onto contemporary
science. But even if it's true that science does not allow explanations
involving non-mechanistic processes, it certainly does allow the action of a
mind to be inferred where no judgement need be made as to whether mental
processes are mechanistic or not (and such a judgement is generally
unnecessary).
An alternative interpretation of Dembski's claim might be that contemporary
science rejects design as a legitimate mode of explanation in accounting for the
origin of biological organisms. If this is what he means, then I reject
the claim. If we were to discover the remains of an ancient alien civilization
with detailed records of how the aliens manipulated the evolution of organisms,
then I think that mainstream science would have little difficulty accepting this
as evidence of design in biological organisms.
The word natural has been the source of much confusion in the debate
over Intelligent Design. It has two distinct meanings: one is the complement of
artificial, i.e. involving intelligent agency; the other is the
complement of supernatural. Dembski tells us that he will use the word in
the former sense: "...I am placing natural causes in contradistinction to
intelligent causes" (p. xiii). He then goes on to say that contemporary science
is wedded to a principle of methodological naturalism:
According to methodological naturalism, in explaining any natural
phenomenon, the natural sciences are permitted to invoke only natural causes
to the exclusion of intelligent causes. [p. xvi]
But the methodological naturalism on which most scientists insist requires
only the rejection of supernatural explanations, not explanations involving
intelligent agency. Indeed, we have just seen that contemporary science allows
explanations involving human designers and, I argue, intelligent alien beings.
Perhaps what Dembski really means is that methodological naturalism rejects the
invocation of an "unembodied designer" (to use his term).6
Dembski introduces the term chance hypothesis to describe proposed
explanations which rely entirely on natural causes. This includes processes
comprising elements of both chance and necessity (p.15), as well as purely
deterministic processes. It may seem odd to refer to purely deterministic
hypotheses as chance hypotheses, but Dembski tells us that "necessity can be
viewed as a special case of chance in which the probability distribution
governing necessity collapses all probabilities either to zero or one" (p.71).
Since Dembski defines design as the complement of chance and necessity, it
follows that a chance hypothesis could equally well (and with greater clarity)
be called a non-design hypothesis. And since he defines natural causes as the
complement of design, we can also refer to chance hypotheses as natural
hypotheses. Dembski's use of the term chance hypothesis has caused considerable
confusion in the past, as many people have taken chance to mean purely random,
i.e. all outcomes being equally probable. While Dembski's usage has been
clarified in No Free Lunch, I believe it still has the potential to
confuse. For the sake of consistency with Dembski's work, I will generally use
the term chance hypothesis, but I will switch to the synonym natural
hypothesis or non-design hypothesis when I think this will increase
clarity.
3. The Chance-Elimination Method
Ignorance, Madam, pure ignorance. Samuel Johnson (on being asked how he came to define a word
incorrectly in his dictionary)
In Chapter 2 of No Free Lunch, Dembski describes a method of inferring
design based on what he calls the Generic Chance Elimination Argument. I'll
refer to this method as the chance-elimination method. This method
assumes that we have observed an event, and wish to determine whether any design
was involved in that event.
The chance-elimination method is eliminative--it relies on rejecting chance
hypotheses. Dembski gives two methods for eliminating chance hypotheses: a
statistical method for eliminating individual chance hypotheses, and
proscriptive generalizations, for eliminating whole categories of chance
hypotheses.
3.1 Dembski's Statistical Method
The fundamental intuition behind Dembski's statistical method is this: we
have observed a particular event (outcome) E and wish to check whether a given
chance hypothesis H provides a reasonable explanation for this outcome.7 We
select an appropriate rejection region (a set of potential outcomes) R,
where E is in R, and calculate the probability of observing an outcome in this
rejection region given that H is true, i.e. P(R|H). If
P(R|H) < α, where α is an appropriate small probability bound,
we consider it implausible that an event of such small probability could have
occurred, and so we reject the chance hypothesis H which gave rise to
this small probability.
It is important to note that we need to combine the probabilities of all
outcomes in an appropriate rejection region, and not just take the probability
of the particular outcome observed, because outcomes can individually have small
probabilities without their occurrence being significant. A rejection region
which is appropriate for use in this way is said to be detachable from
the observed outcome, and a description of a detachable rejection region is
called a specification (though Dembski often uses the terms rejection
region and specification interchangeably).
Consider Dembski's favourite example, the Caputo case (pp. 55-58). A Democrat
politician, Nicholas Caputo, was responsible for making random draws to
determine the order in which the two parties (Democrat and Republican) would be
listed on ballot papers. Occupying the top place on the ballot paper was known
to give the party an advantage in the election, and it was observed that in 40
out of 41 draws Caputo drew a Democrat to occupy this favoured position. In 1985
it was alleged that Caputo had deliberately manipulated the draws in order to
give his own party an unfair advantage. The court which considered the
allegation against Caputo noted that the probability of picking his own party 40
out of 41 times was less than 1 in 50 billion, and concluded that "confronted
with these odds, few persons of reason will accept the explanation of blind
chance."8
In conducting his own analysis of this event, Dembski arrives at the same
probability as did the court, and explains the reasoning behind his conclusion.
The chance hypothesis H which he considers is that Caputo made the draws
fairly, with each party (D and R) having a 1/2 probability of being selected for
the top place on each occasion.
Suppose that we had observed a typical sequence of 41 draws, such as the
following:
DRRDRDRRDDDRDRDDRDRRDRRDRRRDRRRDRDDDRDRDD
The probability of this precise sequence occurring, given H, is
extremely small: (1/2)41 = 4.55 × 10-13. However, unless
that particular sequence had been predicted in advance, we would not consider
the outcome at all exceptional, despite its low probability, since it was very
likely that some such random looking sequence would occur. The historical
sequence, on the other hand, contained just one R, and so looked something like
this:
DDDDDDDDDDDDDDDDDDDDDDRDDDDDDDDDDDDDDDDDD
The second sequence (call it E) has exactly the same probability as the first
one, i.e. P(E|H) = 4.55 × 10-13, but this time we would
consider it exceptional, because the probability of observing so many Ds is
extremely small. Any outcome showing as many Ds as this (40 or more Ds out of 41
draws) would have been considered at least as exceptional, so the probability we
are interested in is the probability of observing 40 or more Ds. "40 or more
Ds", then, is our specification, and, as it happens, there are 42 different
sequences matching this specification, so P(R|H) = 42 ×
P(E|H) = 1.91 × 10-11, or about 1 in 50 billion. In
other words, the probability we are interested in here is not the probability of
the exact sequence we observed, but the probability of observing some outcome
matching the specification. If we decide that this probability is small enough,
we reject H, i.e. we infer that Caputo's draws were not fair. From now
on, I will use the expression "small probability" to mean "probability below an
appropriate probability bound".
In order to apply Dembski's method, we need to know how to select an
appropriate specification and probability bound. Dembski expounds at length a
set rules for selecting these parameters, but they can be boiled down to the
following:
-
An appropriate specification is merely any one which can be derived (in
some loose sense) from background knowledge which was available to us before
observing the event in question. For example, when Dembski applies his method
to the bacterial flagellum--his only biological example--he doesn't bother to
use the technical rules that he developed earlier, or even to state the
specification explicitly. Reading between the lines, his specification appears
to be "anything with the function of an outboard rotary motor", and the only
justification he gives for this specification is the statement that "humans
developed outboard rotary motors well before they figured out that the
flagellum was such a machine" (p. 289).
-
Dembski distinguishes between local and universal probability
bounds. A local probability bound is one which is calculated for the purpose
of a particular statistical test.9 The
procedure for calculating such a bound is difficult and highly arbitrary (p.
83), so Dembski generally falls back on his universal probability bound. This
is a very small number, 10-150 (i.e. 1 in 10150), which
Dembski tells us is the smallest probability bound we need ever use, and which
we can always use in the absence of a suitable local probability bound. He
calculates it by multiplying the number of elementary particles in the
Universe, the maximum possible number of elementary particle transitions (the
inverse of the Planck time) per second, and the number of seconds in a billion
times the current age of the Universe, to give a figure which, he argues, is
the maximum number of probabilistic resources we need ever consider (p.
22):
1080 × 1045 × 1025 =
10150
Although I believe Dembski's statistical method is seriously flawed, the
issue is not important to my refutation of Dembski's design inference. For the
remainder of the main body of this critique, therefore, I will assume for the
sake of argument that the method is valid. A discussion of the flaws will be
left to an appendix. It is
worth noting, however, that this method has not been published in any
professional journal of statistics and appears not to have been recognized by
any other statistician.
3.2 Proscriptive Generalizations
Dembski argues that we can eliminate whole categories of chance hypotheses by
means of proscriptive generalizations. For example, he mentions the
second law of thermodynamics, which proscribes the possibility of a perpetual
motion machine. He describes the logic of such generalizations in terms of
mathematical invariants (p. 274), though this adds absolutely nothing to
his argument.
I accept that proscriptive generalizations can sometimes be made, and Dembski
is welcome to use them to eliminate specific categories of chance hypotheses.
But there is no proscriptive generalization that can rule out all chance
hypotheses. Furthermore, his claim to have found a proscriptive generalization
against Darwinian evolution of irreducibly complex systems is hollow (see 4.2 below).
3.3 The Argument From Ignorance
The conclusion of the Generic Chance Elimination Argument (step #8) is stated
by Dembski as follows:
S [the subject making the inference] is warranted in inferring
that E [the observed outcome] did not occur according to any of the chance
hypotheses in {Hi}i in I and therefore that E
exhibits specified complexity. [p. 73]
{Hi} is the set of all chance hypotheses which we believe
"could have been operating to produce E" (p.72). Dembski also writes:
But what happens once some causal mechanism is found that accounts
for a given instance of specified complexity? Something that is specified and
complex is highly improbable with respect to all causal mechanisms currently
known. Consequently, for a causal mechanism to come along and explain
something that previously was regarded as specified and complex means that the
item in question is in fact no longer specified and complex with respect to
the newly found causal mechanism. [p. 330]
So, when we have eliminated all the chance hypotheses we
can think of, we infer that the event was highly improbable with respect to all
known causal mechanisms, and we call this specified complexity. Later
Dembski tells us that an inference of specified complexity should lead
inevitably to an inference of design. This being the case, it's not clear that
the notion of specified complexity is serving any useful purpose here. Why not
cut out the middleman and go straight from the Generic Chance Elimination
Argument to design? Unfortunately, the introduction of this middleman does serve
to cause considerable confusion, because Dembski equivocates between this sense
of specified complexity and the sense assigned by his uniform-probability method
of inference (which I will explain in section 6). To help
clear up the confusion, I will refer to this middleman sense as eliminative
specified complexity and to the other sense as uniform-probability
specified complexity. Note that Dembski's specified complexity is not a
quantity: an event simply exhibits specified complexity or it doesn't.
Thus we see that the chance-elimination method is purely eliminative. It
tells us to infer design when we have ruled out all the chance (i.e. non-design)
hypotheses we can think of. The design hypothesis says nothing whatsoever about
the identity, nature, aims, capabilities or methods of the designer. It just
says, in effect, "a designer did it".10
This type of argument is commonly known as an argument from ignorance
or god-of-the-gaps argument. So there is no danger of misunderstanding,
let me clarify that the accusation of argument from ignorance is not an
assertion that those making the argument are ignorant of the facts, or even that
they are failing to utilize the available facts. The proponents of an argument
from ignorance are demanding that their explanation be accepted just because the
scientific community is ignorant (at least partially) of how an event occurred,
rather than because their own explanation has been shown to be a good one. Note
that an argument from scientific ignorance differs from the deductive fallacy of
argument from ignorance. The deductive fallacy takes the following form: "My
proposition has not been proven false, so it must be true." The scientific
argument from ignorance is not a deductive fallacy, because scientific
inferences are not deductive arguments.
A god-of-the-gaps argument is an argument from ignorance in which the default
hypothesis, to be accepted when no alternative hypothesis is available, is "God
did it". Since Dembski tells us that his criterion only infers the action of an
unknown designer, and not necessarily a divine one, the term
designer-of-the-gaps might be more appropriate here, but I think it is
reasonable to use the more familiar term, since the arguments follow the same
eliminative pattern and Dembski has made it clear that the designer he has in
mind is the Christian God. The god-of-the-gaps argument should not be
confused with a god-of-the-gaps theology. The latter proposes that God's
actions are restricted to those areas of which we lack knowledge, but does not
offer this as an argument for the existence of God.
Dembski makes no good case for awarding such a privileged status to the
design hypothesis. Why should we prefer "an unknown designer did it" to "unknown
natural causes did it" or "we don't know what did it"? Furthermore, as we shall
see, he tells us to accept design by elimination even when we do have some
outline ideas for how natural causes might have done it.
3.4 Dembski's Responses to the Charge of Argument From
Ignorance
Since arguments from ignorance are almost universally rejected as unsound by
scientists and philosophers of science, Dembski is sensitive to the charge, but
his attempts to avoid facing up to the obvious are mere evasions.
In response to this criticism, note first that even though
specified complexity is established via an eliminative argument, it is not
fair to say that it is established via a purely eliminative argument.
If the argument were purely eliminative, one might be justified in saying that
the move from specified complexity to a designing intelligence is an argument
from ignorance (i.e., not X therefore Y). But unlike Fisher's approach to
hypothesis testing, in which individual chance hypotheses get eliminated
without reference to the entire set of relevant chance hypotheses that might
explain a phenomenon, specified complexity presupposes that the entire set of
relevant chance hypotheses has first been identified. This takes considerable
background knowledge. What's more, it takes considerable background knowledge
to come up with the right pattern (i.e., specification) for eliminating all
those chance hypotheses and thus for inferring design. [p. 111]
Dembski is misconstruing the charge of argument from ignorance. It is not a
question of how much knowledge we have utilized. Scientific knowledge is always
incomplete. The chance-elimination method is purely eliminative because it makes
no attempt to consider the merits of the design hypothesis, but merely relies on
eliminating the available alternatives.
Design inferences that infer design by identifying specified
complexity are therefore not purely eliminative. They do not merely exclude,
but they exclude from an exhaustive set in which design is all that remains
once the inference has done its work (which is not to say that the set is
logically exhaustive; rather, it is exhaustive with respect to the inquiry in
question--that is all we can ever do in science). Design inferences, by
identifying specified complexity, exclude everything that might in turn
exclude design. [p. 111]
Dembski's phrase "exhaustive with respect to the inquiry in question" is the
sort of circumlocution in which he excels. It just means that the set is as
exhaustive as we can make it. In other words, it's a fancy way to say we have
eliminated all the chance hypotheses we could think of.
Design inferences therefore eliminate chance in the global sense
of closing the door to all relevant chance explanations. To be sure, this
cannot be done with absolute finality since there is always the possibility
that some crucial probability distribution was missed. Nonetheless, it is not
enough for the design skeptic merely to note that adding a new chance
explanation to the mix can upset a design inference. Instead, the design
skeptic needs to explicitly propose a new chance explanation and argue for its
relevance to the case at hand. [pp. 67-68]
This is a clear argument from ignorance. Unless design skeptics can propose
an explicit natural explanation, Dembski tells us, we should infer design.
For any event whatsoever, there exists a probability distribution
that concentrates all probability on that event and thus assigns it a
probability of one. It therefore makes no sense to criticize my generalization
of Fisher's approach to hypothesis testing for failing to consider all
possible chance hypotheses. [p. 70]
Dembski is not being criticized for failing to eliminate all possible chance
hypotheses, but for adopting a purely eliminative method in the first place.
Archeologists infer that certain chunks of rock are arrowheads.
Detectives infer that certain deaths were deliberate. Cryptographers infer
that certain random looking symbol strings are actually encrypted messages. In
every case they might be wrong, and further knowledge might reveal a plausible
chance hypothesis behind what originally appeared to be designed. But such
sheer possibilities by themselves do nothing to overturn our confidence in
design inferences. [p. 71]
Yes, these design inferences are fallible, as are all scientific inferences.
That is not the issue. The difference is that these inferences are not purely
eliminative. The experts in question have in mind a particular type of
intelligent designer (human beings) of which they know much about the abilities
and motivations. They can therefore compare the merits of such an explanation
with the merits of other explanations.
If Dembski wishes to defend god-of-the-gaps arguments as a legitimate mode of
scientific inference, he is welcome to try. What is less welcome are his
attempts to disguise his method as something more palatable.
3.5 Comparative and Eliminative Inferences
One way in which Dembski attempts to defend his method is to suggest that
there is no viable alternative. The obvious alternative, however, is to consider
all available hypotheses, including design hypotheses, on their merits, and then
select the best of them. This is the position adopted by almost all philosophers
of science, although they disagree on how to evaluate the merits of hypotheses.
There seems no reason to treat inferences involving intelligent agents
differently in this respect from other scientific inferences.
Dembski argues at some length against the legitimacy of comparative
approaches to inference (pp. 101-110, 121n59). I will not address the specifics
of the likelihood approach, on which he concentrates his fire. I leave that to
its proponents. However, his rejection of comparative inferences altogether is
clearly untenable. When we have two or more plausible hypotheses
available--whether those involve intelligent agents or not--we must use some
comparative method to decide between them.
Consider, for example, the case of the archaeologists who make inferences
about whether flints are arrowheads made by early humans or naturally occurring
pieces of rock. Let us take a borderline case, in which a panel of
archaeologists is divided about whether a given flint, taken from a site
inhabited by early humans, is an arrowhead. Now suppose that the same panel had
been shown the same flint but told that it came from a location which has never
been inhabited by flint-using humans, say Antarctica. The archaeologists would
now be much more inclined to doubt that the flint was man-made, and more
inclined to attribute it to natural causes. A smaller proportion (perhaps none
at all) would now infer design. The inference of design, then, was clearly
influenced by factors affecting the plausibility of the design hypothesis:
whether or not flint-using humans were known to have lived in the area. The
inference was not based solely on the elimination of natural hypotheses.
It is not my intention to argue for any particular method of comparing
hypotheses. Philosophers of science have proposed a number of comparative
approaches, usually involving some combination of the following criteria:
- Likelihood. The probability of the evidence occurring given the hypothesis
in question.
- Prior probability or plausibility. Our degree of belief in the hypothesis
prior to observing the evidence, or assuming we had not observed it.
- Predictive power. The degree to which the hypothesis determines which
potential observations are possible (or probable) and which are impossible (or
improbable).
- Falsifiability. The degree to which the hypothesis "risks" being falsified
by new evidence.
- Parsimony. The degree to which the hypothesis observes the principle of
Occam's razor: "Do not multiply entities needlessly."11
Other criteria often cited include explanatory power, track record, scope,
coherence and elegance.
In opposing comparative methods, Dembski argues that hypotheses can be
eliminated in isolation without there necessarily being a superior competitor.
In practical terms, I agree, although I suspect that we would not eliminate a
hypothesis unless we had in the back of our minds that there existed a plausible
possibility of a better explanation. I do not deny that we can
eliminate a hypothesis without having a better one in mind; I deny that
we can accept a hypothesis without having considered its merits, as
Dembski would have us do in the case of his design hypothesis. If all the
available hypotheses score too badly according to our criteria, it may be best
to reject all of them and just say "we don't know".
3.6 Reliability and Counterexamples
Dembski argues, on the basis of an inductive inference, that the
chance-elimination method is reliable:
First (section 1.6) I offered an inductive argument, showing that
in all cases where we know the causal history and where specified complexity
was involved, an intelligence was involved as well. The inductive
generalization that follows is that all cases of specified complexity involve
intelligence. [p. 110]
Setting aside the question of whether such an induction would be justified if
its premise were true, let's just consider whether or not the premise is true.
Contrary to Dembski's assertion, his section 1.6 did not show anything of the
sort. In fact, the only cases where we know that Dembski's method has been used
to infer design are the two examples that Dembski himself describes: the Caputo
case and the bacterial flagellum. And in neither of these cases has design been
independently established.
Dembski wants us to believe that his method of inference is basically the
same method already used in our everyday and scientific inferences of design. I
have already argued that this is untrue. But even if we suppose, for the sake of
argument, that our typical design inferences are indeed based on the sort of
purely eliminative approach proposed by Dembski, then it is not difficult to
find counterexamples, in which design was wrongly inferred because of ignorance
of the true natural cause:
-
Fairy rings. These are rings of mushrooms caused by a fungus spreading
through grass at a uniform rate from a given starting point. Mushrooms are
manifested on the outer rim of the affected circle. Before the cause was
known, these rings were often attributed to intelligent designers ("fairies").
If we take the chance hypothesis that the mushrooms were randomly located
throughout a meadow (with a uniform probability distribution), the probability
of them forming a neat circle is clearly small enough to justify the rejection
of this hypothesis (by use of an appropriate local probability bound, if not
Dembski's universal probability bound).12
Using Dembski's chance-elimination method, specified complexity (and hence
design) would have been inferred erroneously.
-
Moon craters. On observing the major craters of the Moon, Johannes Kepler
concluded that they were too circular to have occurred by chance, and so must
have been created by inhabitants of the Moon. If we take the chance hypothesis
that the craters were formed out of many individual hills, and that these
hills were randomly distributed across the surface of the Moon, then the
probability of them forming such good circles is clearly small enough to
reject this hypothesis. Using Dembski's chance-elimination method, specified
complexity (and hence design) would have been inferred, but we now know that
these craters have a natural explanation--impacts from falling objects. I am
indebted for this example to Dembski himself, who describes it13
but fails to notice that it provides a counterexample to his
claim.
Perhaps Dembski would object that his claim ("in all cases where we know the
causal history and where specified complexity was involved, an intelligence was
involved as well") was only referring to cases where we observe specified
complexity today. But, by definition, those are cases where we don't have
a plausible natural explanation. If we had one, we would not infer specified
complexity. If we know the causal history and it was not a natural cause, it
must have been design. So, if this is what Dembski means, his claim is a
tautology. It says that, whenever the cause is known to be design, the cause is
design! You cannot make an inductive inference from a tautology.
It would do Dembski no good to claim that these are cases of derived
design (see 6.1
below), e.g. that mushrooms and the solar system were originally designed.
The chance-elimination method infers design in the particular event which is
alleged to have small probability of occurring under natural causes. For
example, in the case of the flagellum, Dembski claims that design was involved
in the origin of the flagellum itself, and not just indirectly in terms of the
Earth or the Universe having been designed.
3.7 The Explanatory Filter
The chance-elimination method is initially introduced in a simplified form
called the Explanatory Filter. The criterion for the filter to recognize
design is labelled the complexity-specification criterion. Unfortunately,
the use of this simplified account has caused considerable confusion in the
past, because it possesses two misleading features:
-
The description of the Explanatory Filter hardly mentions the concept of
chance hypotheses, and implies that we need consider only one probability
distribution. The flow chart for the filter (p. 13) should contain a loop, to
be executed for each chance hypothesis. Many readers of Dembski's past work
have been led to the erroneous conclusion that we only need to calculate the
probability with respect to a uniform probability distribution.
-
The Explanatory Filter has separate nodes for complexity (which Dembski
uses here as a synonym for improbability) followed by specification, as if
these were two separate criteria. But, as we saw above, we cannot calculate
the probability until we have formulated a specification. Many of Dembski's
readers in the past have erroneously interpreted the filter as follows: note
that the observed outcome is specified (in some sense) and then calculate the
probability of that single outcome (when they should have calculated the
probability of an entire rejection region).
Although Dembski has made some attempts to clarify the situation in No
Free Lunch, his continued use of the Explanatory Filter in its highly
misleading form is inexplicable. And the misdirection is not limited to the
Explanatory Filter itself. It occurs elsewhere too, in statements such as
this:
Determining whether an irreducibly complex system exhibits
specified complexity involves two things: showing that the system is specified
and calculating its probability... [p. 289]
4. Applying the Method to Nature
He uses statistics as a drunken man uses lampposts--for
support rather than illumination. Andrew Lang
(1844-1912), poet and novelist
4.1 A Tornado in a Junkyard
It has been several years since Dembski first claimed to have detected design
in biology by applying his method of inference. Yet until the publication of
No Free Lunch, he had never provided or cited the details of any such
application. Critics were therefore looking forward to seeing the long-promised
probability calculation that would support the claim. While I, for one, did not
expect a convincing calculation, even I was amazed to discover that Dembski has
offered us nothing but a variant on the old Creationist "tornado in a
junkyard"14 straw
man, namely the probability of a biological structure occurring by purely random
combination of components.
The only biological structure to which Dembski applies his method is the
flagellum of the bacterium E. coli. As his method requires him to start
by determining the set {Hi}of all chance hypotheses which
"could have been operating to produce E [the observed outcome]" (p. 72), one
might expect an explicit identification of the chance hypothesis under
consideration. Dembski provides no such explicit identification, and the reader
is left to infer it from the details of the calculation. Perhaps the reason
Dembski failed to identify his chance hypothesis is that, when clearly named, it
is so transparently a straw man. No biologist proposes that the flagellum
appeared by purely random combination of proteins--they believe it evolved by
natural selection--and all would agree that the probability of appearance by
random combination is so minuscule that this is unsatisfying as a scientific
explanation. Therefore for Dembski to provide a probability calculation based on
this absurd scenario is a waste of time. There is no need to consider whether
Dembski's calculation is correct, because it is totally irrelevant to the issue.
Nevertheless, since Dembski does not state clearly that he has based his
calculation on a hypothesis of purely random combination, I will describe the
calculation briefly in order to demonstrate that this is the case.
Dembski tells us to multiply three partial probabilities to arrive at the
probability of a "discrete combinatorial object":
pdco = porig ×
plocal × pconfig
-
plocal is the probability of a suitable collection of
proteins being drawn from a set of existing proteins which includes the ones
required. Dembski assumes that the proteins are randomly drawn from among the
4289 proteins coded for by E. coli's DNA, that 5 copies are needed of
each of 50 different proteins (making 250 proteins altogether), and that, in
each case, there are 10 different proteins that would be acceptable (i.e.
there are 9 possible substitutes for the real protein. In effect, we have to
make 250 draws, and at each draw we have a 500/4289 probability of picking a
useful protein, giving an overall probability of (500/4289)250.
-
pconfig is the probability that, given the right
collection of proteins, they will form a viable flagellum if arranged at
random. Dembski aims to draw from a uniform probability distribution
over all the possible ways of arranging the selected proteins:
Strictly speaking, the configuration probability for a discrete
combinatorial object that exhibits some function is the ratio of all the
ways of arranging its building blocks that preserve the function divided by
all the possible ways whatsoever of arranging the building blocks. [pp.
294-295]
Since he can't calculate this directly, he uses an approximation that he
calls a perturbation probability. We need not concern ourselves with
the details.
-
porig is the probability of all the individual proteins
forming by random combination of amino acids, and is again based on a
perturbation probability.
Each of these probabilities individually is below Dembski's universal
probability bound, so he does not proceed to multiply them.
Incidentally, Dembski errs in choosing to calculate a formation probability
for the flagellum itself. He should have considered the formation of the DNA to
code for a flagellum. If a flagellum appeared without the DNA to code for it, it
would not be inherited by the next generation of bacteria, and so would be
lost.
4.2 Irreducible Complexity
In order to justify his failure to calculate the probability of the flagellum
arising by Darwinian evolution, Dembski invokes the notion of irreducible
complexity, which, he argues, provides a proscriptive generalization against
Darwinian evolution of the flagellum. Irreducible complexity was introduced into
the Intelligent Design argument by biochemist Michael Behe. The subject has been
addressed in great detail elsewhere, so I will not repeat all the objections.15
However, I would like to draw attention to a point which some readers of Behe
have overlooked. Behe divided potential Darwinian pathways for the evolution of
an irreducibly complex (hereafter IC) system into two categories: direct
and indirect.16 The
direct pathways are those in which a system evolves purely by the
addition of several new parts that provide no advantage to the system until all
are in place. All other potential pathways are referred to as indirect.
Behe then argues that IC systems cannot evolve via direct pathways. But his
direct pathways exclude two vital elements of the evolutionary process: (a) the
evolution of individual parts of a system; and (b) the changing of a system's
function over time, so that, even though a given part may have contributed
nothing to the system's current function until the other parts were in place, it
may well have contributed to a previous function. When it comes to indirect
pathways, Behe has nothing but an argument from ignorance: no one has given a
detailed account of such a pathway. The truth of this assertion has been
contested, but it depends on just how much detail is demanded. Behe demands a
great deal. He then asserts that the evolution of an IC system by indirect
pathways is extremely improbable, but he has provided no argument to support
this claim. It is merely his intuition.17
Dembski repeats the claim that the problem of explaining the evolution of IC
molecular systems has "proven utterly intractable" (p. 246), but evolutionary
explanations have now been proposed for several of the systems cited by Behe,
including the blood-clotting cascade, the immune system, the complement system
and the bacterial flagellum. The last of these is highly speculative, but is
sufficient to refute the claim of utter intractability.18
What then has Dembski added to the debate over irreducible complexity? First,
he has attempted to counter the objections of Behe's critics. I won't comment on
these except to say that some of these critics appear to have misunderstood what
Behe meant by irreducible complexity. This is unsurprising since his definition
was vague and was accompanied by several misleading statements. Indeed, Behe
himself has admitted that his definition was ambiguous.19 He
has even tentatively proposed a completely new definition.20
Second, Dembski has proposed a new definition of his own, making three major
changes:
-
Behe was very vague about how a system should be divided into parts.
Sometimes he took individual proteins as his parts, but in the case of the
bacterial flagellum he divided the system into just three parts, "a paddle, a
rotor, and a motor", each consisting of multiple proteins (Darwin's Black
Box, p. 72). Dembski requires the parts to be "nonarbitrarily
individuated" (p. 285), which doesn't tell us much. What is significant,
however, is that in the case of the bacterial flagellum he chooses individual
proteins as his parts. In fact, he seems not to have even noticed that Behe
divided the flagellum into only three parts:
Behe shows that the intricate machinery in this molecular
motor--including a rotor, a stator, O-rings, bushings, and a drive
shaft--requires the coordinated interaction of about thirty proteins and
another twenty or so proteins to assist in their assembly. Yet the absence
of any one of these proteins would result in the complete loss of motor
function.... But a flagellum without its full complement of protein parts
does not function at all. Behe therefore concludes that if the Darwinian
mechanism is going to produce the flagellum, it will have to do so in one
generation. [pp. 249-251]
-
Whereas Behe only considered a system to be IC if all of its parts
were indispensable, Dembski considers a system IC if it has an irreducible
core of indispensable parts.
-
Dembski has added two new conditions which must be met before a system can
be considered evidence of intelligent design. In addition to being IC, the
system's irreducible core must possess "numerous and diverse parts" and have
the property of "minimal complexity and function" (p. 287). Both of these
conditions are rather vague. "Numerous" and "diverse" are not quantified. The
complexity of the system apparently need not be quite minimal, since, in the
case of the bacterial flagellum, Dembski argues only that "the complexity of
known flagella is not very different from the minimal complexity that
such systems might in principle require" (p. 288, my emphasis).
The last of these changes is sure to create yet more confusion. It is no
longer enough, according to Dembski, to show that a system is IC. It must also
meet the two additional criteria. Yet, elsewhere in his book, Dembski continues
to refer to irreducible complexity as a sufficient condition for inferring
design:
In particular, the claim that the Darwinian mechanism can account
for the full diversity of living forms will have to be rejected inasmuch as
this mechanism is unable to generate the specified complexity inherent in--to
take the most popular example--irreducibly complex biochemical systems (see
chapter 5). [p. 324]
I can understand the temptation to use irreducibly complex as a
shorthand term for irreducibly complex with an irreducible core which has
numerous and diverse parts and exhibits minimal complexity and function, but
Dembski should really have introduced a new term for the latter. From now on,
when claiming to have found an example of irreducible complexity in nature,
Intelligent Design proponents should specify which of the following definitions
they have in mind: Behe's original definition; Behe's corrected version of his
original definition; Behe's proposed new definition; Dembski's definition; or
Dembski's definition plus the two additional criteria. I predict most will fail
to do so. For the remainder of this article, I will use the term IC in the last
of these senses. It should not be assumed that all the examples of IC systems
offered by Behe necessarily meet Dembski's criteria. Dembski considers only the
bacterial flagellum. Whether Behe's other example systems are IC in this new
sense remains to be established.
Let us accept, for the sake of argument,
that Dembski's definition is tight enough to ensure that IC systems cannot
evolve by direct pathways. What has he said on the vital subject that
Behe failed to address--the subject of indirect pathways? The answer is
nothing. The crux of his argument is this:
To achieve an irreducibly complex system, the Darwinian mechanism
has but two options. First, it can try to achieve the system in one fell
swoop. But if an irreducibly complex system's core consists of numerous and
diverse parts, that option is decisively precluded. The only other option for
the Darwinian mechanism then is to try to achieve the system gradually by
exploiting functional intermediates. But this option can only work so long as
the system admits substantial simplifications. The second condition [that the
irreducible core of the system is at the minimal level of complexity needed to
perform its function] blocks this other option. Let me stress that there is no
false dilemma here--it is not as though there are other options that I have
conveniently ignored but that the Darwinian mechanism has at its disposal.[p.
287]
But there is indeed an option that Dembski has overlooked. The system could
have evolved from a simpler system with a different function. In that
case there could be functional intermediates after all. Dembski's mistake is to
assume that the only possible functional intermediates are intermediates having
the same function.
Dembski's failure to consider the possibility of a change of function is seen
in his definition of irreducible complexity:
Definition ICfinal--A system performing a given
basic function is irreducibly complex if it includes a set of
well-matched, mutually interacting, non-arbitrarily individuated parts such
that each part in the set is indispensable to maintaining the system's basic,
and therefore original, function. The set of these indispensable parts is
known as the irreducible core of the system. [p. 285]
There is no reason why a system's basic function should be its original one.
The concepts of basic function and original function may not even be
well-defined. If a system performs two vital functions, which is the basic one?
The concept of an original function assumes there is an identifiable time at
which the system came into existence. But the system may have a long history in
which parts have come and gone, and functions have changed, making it impossible
to trace back the origin of the system to one particular time. And what is a
system? If two proteins start to interact in a beneficial way , do they
immediately become a system? If so, we may have to trace the history of a system
all the way back to the time when one it was just two interacting proteins.
There is a tendency among antievolutionists to think of biological systems as
if they were like man-made machines, in which the system and its parts have been
designed for one specific function and are difficult to modify for another
function. But biological systems are much more flexible and dynamic than
man-made ones.
A few other points are worth noting:
-
Changes of function are not an ad hoc idea thought up as a
last-ditch attempt to solve a nasty problem. They are a fundamental feature of
evolution. New systems do not just appear out of nowhere. Most systems will
have evolved from an earlier system having a different function.
-
Changes of function can occur in two ways. First, a mutation may create a
new capability. Second, a change in the environment may provide a new use for
a system, e.g. a fish's fin starts to be used as a primitive leg in shallow
water. In either case, the system may perform the new function very poorly at
first, subsequently mutating to perform it better. Behe and Dembski both
emphasize how well coordinated the parts of a system seem to be. But they may
have been far less well coordinated in the past.
-
A system may have more than one function. In the example above, the fish's
fin may continue to be used for swimming as well as clambering over submerged
rocks.
-
There is no clear distinction between systems and parts. Any functional
structure can be considered both a system in its own right and a part of a
larger system. So we need not think in terms of a system acquiring a large
number of parts consisting of individual proteins, as Dembski would have us
do. A system may instead acquire a small number of sub-systems, each
consisting of multiple proteins.
-
Instead of an IC system having to arise by the simultaneous combination of
many parts, we now see that it can arise by the gradual acquisition of a few
parts. This no longer sounds as unlikely as Behe and Dembski made it
seem.
Before finishing this section, it might be useful to clear up a few more red
herrings which Dembski introduces into his discussion of irreducible
complexity.
-
Causal specificity. This is just another cover for the argument from
ignorance:
Unless a concrete model is put forward that is detailed enough
to be seriously criticized, then it is not going to be possible to determine
the adequacy of that model. This is of course another way of saying that the
scaffolding objection has yet to demonstrate causal specificity when applied
to actual irreducibly complex biochemical systems. [p. 254]
In other words, until a sufficiently detailed natural hypothesis is
provided, we should go ahead and infer design. It doesn't bother Dembski (or
Behe who makes the same point) that their alternative hypothesis (design)
lacks any details whatsoever.
-
Invariants. Dembski describes some geometrical problems which have
no solution, and explains how the non-existence of a solution can be proven by
showing that a certain property is invariant under transformation of the
system. How is this relevant to irreducible complexity? Does Dembski use the
invariance of some property to establish that IC systems cannot evolve? No,
the property he claims to be invariant (under natural evolution) is the
property of irreducible complexity itself. But the assertion that irreducible
complexity cannot be produced by natural evolution was exactly the point which
he was trying to establish. In other words, invariance does no work in
establishing Dembski's conclusion. It is just another way of expressing that
conclusion.
In trying to relate the subject of invariants to evolution, Dembski writes:
"think of an effective invariant here as an insurmountable obstacle for the
Darwinian mechanism" (p. 285). One has to wonder why he does not just use the
expression "insurmountable obstacle" from the start, and skip the whole
irrelevant discussion of invariants.
-
Specified complexity. Dembski likes to say that "irreducible
complexity is a special case of specified complexity" (p. 289), as if this
demonstrated the integration of two concepts into a coherent framework. But we
have already seen that specified complexity is merely a label we apply when we
have no plausible natural hypothesis to explain some event. So, to say that
irreducible complexity is a case of specified complexity is just another way
to repeat the claim that we have no natural explanation for the origin of the
bacterial flagellum (which is the only biological system Dembski has shown to
be IC in his sense).
5. Evolutionary Algorithms
Attempt the end, and never stand to doubt; Nothing's so
hard, but search will find it out. Robert Herrick
(1591-1674)
In recent years there has been a considerable growth of interest in
evolutionary algorithms, executed on computers, as a means for solving
optimization problems. As the name suggests, evolutionary algorithms are based
on the same underlying principles as biological evolution: reproduction with
random variations, and selection of the "fittest". Since they appear to
demonstrate how unguided processes can produce the sort of functional
complexity21 that
we see in biology, they are a problem which Dembski needs to address. In
addition, he tries to turn the subject to his advantage, by appealing to a set
of mathematical theorems, known as the No Free Lunch theorems, which place
constraints on the problem-solving abilities of evolutionary algorithms.
5.1 Black-Box Optimization Algorithms
We will be concerned here with a type of algorithm know as a black-box
optimization (or search) algorithm. Such algorithms include evolutionary
algorithms, but are not limited to them. The problems which black-box
optimization algorithms solve have just two defining attributes: a phase
space, and a fitness function defined over that phase space. In the
context of these algorithms, phase spaces are usually called search
spaces. Also the term fitness function is usually reserved for
evolutionary algorithms, the more general term being objective function
or cost function (maximizing an objective function is equivalent to
minimizing a cost function). But I will adopt Dembski's terminology for the sake
of consistency.
The phase space is the set of all potential solutions to the problem. It is
generally a multidimensional space, with one dimension for each variable
parameter in the solution. Most real optimization problems have many parameters,
but, for ease of understanding, it is helpful to think of a two-dimensional
phase space--one with two parameters--which can be visualized as a horizontal
plane. The fitness function is a function over this phase space; in other words,
for every point (potential solution) in the phase space the fitness function
tells us the fitness value of that point. We can visualize the fitness function
as a three-dimensional landscape where the height of a point represents its
fitness (figure 1). Points on hills represent better solutions while points in
valleys represent poorer ones. The terms fitness function and fitness
landscape are used interchangeably.
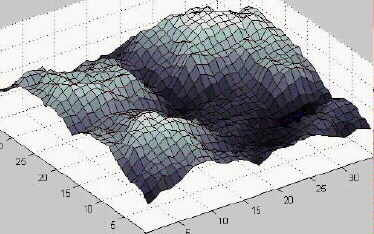
Figure 1. A Fitness Landscape
An optimization algorithm is, broadly speaking, an algorithm for finding high
points in the landscape. Being a black-box algorithm means that it has no
knowledge about the problem it is trying to solve other than the underlying
structure of the phase space and the values of the fitness function at the
points it has already visited. The algorithm visits a sequence of points
(x1, x2, ..., xm), evaluating the fitness,
f(xi), of each one in turn before deciding which point to visit next.
The algorithm may be stochastic, i.e. it may incorporate a random element in its
decisions.
Evaluating the fitness function is typically a very computation-intensive
process, possibly involving a simulation. For example, if we are trying to
optimize the design of a road network, we might want the algorithm to run a
simulation of daily traffic for each possible design that it considers. The
performance of the algorithm is therefore measured in terms of the number of
fitness function evaluations (m) needed to reach a given level of fitness, or
the level of fitness reached after a given number of function evaluations. Each
function evaluation can be thought of as a time step, so we can think in terms
of the level of fitness reached in a given time. Note that we are interested in
the best fitness value found throughout the whole time period, and not just the
fitness of the last point visited.
There are three types of optimization algorithm of interest to us here:
-
Random search (also known as random sampling). This algorithm
just selects each point at random (with a uniform probability distribution)
out of all the points in the phase space.
-
Hill-climbers. A hill-climber visits some or all the points near to
its current location, and moves to the highest one it finds. It never moves
downwards. If it reaches the top of a hill, it gets stuck there, or it may
begin a random search in the hope of finding a higher hill.
-
Evolutionary algorithms. An evolutionary algorithm maintains a
population of individuals (usually randomly generated initially), that evolves
according to rules of selection, recombination, mutation and survival. Each
individual corresponds to a point in the phase space. A shared "environment"
determines the fitness of each individual in the population. The fittest
individuals are more likely to be selected for reproduction (retention or
duplication), while recombination and mutation modify those individuals,
yielding potentially superior ones.
Dembski adopts a very broad definition of evolutionary algorithm which
includes all the optimization algorithms which we consider here,
including random search (pp. 180, 229n9, 232n31).
Another term used by Dembski is blind search. He uses it in two
senses. First it means a random walk, an algorithm which moves from one
location in the phase space to another location selected randomly from nearby
points (p. 190). Later he uses it to mean any search in which the fitness
function has only two possible values: the point being evaluated either is or is
not in a target area (p. 197). The usual (though not exclusive) meaning of
blind search in the literature of evolutionary algorithms is as a synonym
for black-box algorithm.22
5.2 Fine-Tuning the Fitness Function
Dembski recognizes that evolutionary algorithms can produce quite innovative
results, but he argues that they can only do so because their fitness function
has been fine-tuned by the programmer. In doing so, he alleges, the programmer
has "smuggled" complex specified information or specified
complexity into the result. (These two terms will be discussed later.)
Even so, there is something oddly compelling and almost magical
about the way evolutionary algorithms find solutions to problems where the
solutions are not like anything we have imagined. A particularly striking
example is the "crooked wire genetic antennas" of Edward Altshuler and Derek
Linden. The problem these researchers solved with evolutionary (or genetic)
algorithms was to find an antenna that radiates equally well in all directions
over a hemisphere situated above a ground plane of infinite extent. Contrary
to expectations, no wire with a neat symmetric geometric shape solves this
problem. Instead, the best solutions to this problem look like zigzagging
tangles. What's more, evolutionary algorithms find their way through all the
various zigzagging tangles--most of which don't work--to one that actually
does. This is remarkable. Even so, the fitness function that prescribes
optimal antenna performance is well-defined and readily supplies the complex
specified information that an optimal crooked wire genetic antenna seems to
acquire for free. [p. 221]
A similar claim is made regarding biological evolution:
Thus I submit that even if Darwinian evolution is the means by
which the panoply of life on earth came to be, the underlying fitness function
that constrains biological evolution would not be a free lunch and not a brute
given, but a finely crafted assemblage of smooth gradients that presupposes
much prior specified complexity. [p. 212]
These claims are based on a fundamental misconception of the role of the
fitness function in an evolutionary algorithm. A fitness function incorporates
two elements:
-
It reflects our objectives. If our aim is to design a bridge, we might need
to decide what weight to give to a number of conflicting objectives such as
traffic capacity, structural integrity, cost and environmental impact.
-
It encapsulates our relevant knowledge about the real world, in order to
evaluate how well a potential solution meets our objectives.
In general, then, the fitness function defines the problem to be solved, not
the way to solve it, and it therefore makes little sense to talk about the
programmer fine-tuning the fitness function in order to solve the problem. True,
there may be some aspects of the problem which are unknown, or where the
programmer decides, for practical reasons, to simplify his model of the problem.
Here the programmer could make decisions in such a way as to improve the
performance of the algorithm. But there is no reason to think that this makes a
significant contribution to the success of evolutionary algorithms.
In one of his articles, Dembski quotes evolutionary psychologist Geoffrey
Miller in support of his claim that the fitness function needs to be
fine-tuned:
And where exactly does design get built into an evolutionary or
genetic algorithm? According to Miller, it gets built into the fitness
function. He writes:
The fitness function must embody not only the engineer's
conscious goals, but also her common sense. This common sense is largely
intuitive and unconscious, so is hard to formalize into an explicit fitness
function. Since genetic algorithm solutions are only as good as the fitness
functions used to evolve them, careful development of appropriate fitness
functions embodying all relevant design constraints, trade-offs and criteria
is a key step in evolutionary engineering.23
But the engineer's goals, constraints, trade-offs, etc, are parameters of the
problem to be solved. They must be carefully chosen to ensure that the
evolutionary algorithm addresses the right problem, not to guide it to the
solution of a given problem, as Miller tells us in the preceding paragraph:
If the fitness function does not realistically reflect the
real-world constraints and demands that the phenotypic designs will face, the
genetic algorithm may deliver a good solution to the wrong problem.24
It is other elements of the evolutionary algorithm which may have to be
carefully selected if the algorithm is to perform well:
The trick in genetic algorithms is to find schemes that do this
mapping from a binary biting to an engineering design efficiently and
elegantly, rather than by brute-force.... The genetic operators copy and
modify the genotypes from one generation to the next.... Getting the right
balance between mutation and selection is especially important.... Finally,
the evolutionary parameters [such as population size and mutation rate]
determine the general context for evolution and the quantitative details of
how the genetic operators work.... Deciding the best values for these
parameters in a given application remains a black art, driven more by blind
intuition and communal tradition than by sound engineering principles.24
A similar point is made by Wolpert and Macready:
Ultimately, of course, the only important question is, "How do I
find good solutions for my given cost function f?" The proper answer to
this question is to start with the given f, determine certain salient
features of it, and then construct a search algorithm, a, specifically
tailored to match those features. The inverse procedure--far more popular in
some communities--is to investigate how specific algorithms perform on
different f's. This inverse procedure is only of interest to the
degree that it helps us with our primary procedure, of going from (features
concerning) f to an appropriate a.25
Perhaps Dembski's confusion on this subject can be explained by his obsession
with Richard Dawkins' Weasel program,26 to
which he devotes a large part of his chapter on evolutionary algorithms. In that
example, invented only to illustrate one specific point, the fitness function
was indeed chosen in order to help the algorithm converge on the solution. That
program, however, was not created to solve an optimization problem. The program
had a specific target point, unlike real optimization algorithms, where the
solution is unknown.
In the case of biological evolution, the situation is somewhat different,
because the evolutionary parameters themselves evolve over the course of
evolution. For example, according to evolutionary theory, the genetic code has
evolved by natural selection. It is therefore not just good luck that the
genetic code is so suited to evolution. It has evolved to be that way.
When Dembski talks about fine-tuning of the fitness function for biological
evolution, what he really means is fine-tuning of the cosmological and
terrestrial initial conditions, including the laws of physics. When these
conditions are a given, as they are for practical purposes, they contribute to
determining the fitness function. But Dembski argues that these conditions must
have been selected from a set of alternative possibilities in order to make the
evolution of life possible. When considered in this way, alternative sets of
initial conditions should properly be considered as elements in another phase
space, and not as part of the fitness function. Dembski sometimes refers to this
as a phase space of fitness functions. One can understand what he means by this,
but it is potentially confusing, not least because the fitness functions for
biological organisms are not fixed, but evolve as their environment evolves.
We see then that Dembski's argument from fine-tuning of fitness functions is
just a disguised version of the well-known argument from fine-tuning of
cosmological and terrestrial initial conditions.27
Dembski lists a catalogue of cosmological and terrestrial conditions which need
to be just right for the origin of life (pp. 210-211). This argument is an old
one, and I won't address it here. The only new twist that Dembski gives to it is
to cast the argument in terms of fitness functions and appeal to the No
Free Lunch theorems for support. That appeal will be considered below, but first
I want to make a couple of observations.
Dembski's two conclusions cannot both be true. On the one hand he is arguing
that the initial conditions were fine-tuned to make natural evolution of life
possible. On the other hand, he is arguing that natural evolution of life
wasn't possible. Not that there's anything wrong with Dembski having two
bites at the cherry. If one argument fails, he can fall back on the other.
Alternatively Dembski might argue that the cosmic designer made the Universe
almost right for the natural evolution of life, but left himself with a
little work to do later.
If Dembski believes that the initial conditions for evolution were designed,
the obvious thing to do would be to try applying his chance-elimination method
to the origin of those conditions. I note that he doesn't attempt to do so.
5.3 The No Free Lunch Theorems
Dembski attempts to use the No Free Lunch theorems (hereafter NFL) of David
Wolpert and William Macready28 to
support his claim that fitness functions need to be fine-tuned. He presumably
considers NFL important to his case, since he names his book after it. However,
I will show that NFL is not applicable to biological evolution, and even for
those evolutionary algorithms to which it does apply, it does not support the
fine-tuning claim. I'll start by giving a brief explanation of what NFL says,
making a number of simplifications and omitting details which need not concern
us here.
NFL applies only to algorithms meeting the following conditions:
-
The algorithm must be a black-box algorithm, i.e. it has no knowledge about
the problem it is trying to solve other than the underlying structure of the
phase space and the values of the fitness function at the points it has
already visited.
-
In principle, there must be a finite number of points in the phase space
and a finite number of possible fitness values. In practice, however,
continuous variables can be approximated by rounding to discrete values.
-
The algorithm must not visit the same point twice. This can be avoided by
having the algorithm keep a record of all the points it has visited so far,
with their fitness values, so it can avoid repeated visits to a point. This
may not be practical in a real computer program, but most real phase spaces
are sufficiently vast that revisits are unlikely to occur often, so we can
ignore this issue.
-
The fitness function may remain fixed throughout the execution of the
program, or it may vary over time in a manner which is independent of the
progress of the algorithm. These two options correspond to Wolpert and
Macready's Theorems 1 and 2 respectively. However, the fitness function may
not vary in response to the progress of the algorithm. In other words, the
algorithm may not deform the fitness landscape.29
The same algorithm can be used with any problem, i.e. on any fitness
landscape, though it won't be efficient on all of them. In terms of a computer
program, we can imagine inserting various alternative fitness function modules
into the program. We can also imagine the set of all possible fitness functions.
This is the vast set consisting of every possible shape of landscape over our
given phase space. If there are S points in the phase space and F possible
values of the fitness function, then the total number of possible fitness
functions is FS, since each point can have any of F values, and we
must allow for every possible permutation over the S points.
We are now in a position to understand what NFL says. Suppose we take an
algorithm a1, measure its performance on every fitness
function in that vast set of possible fitness functions, and take the average
over all those performance values. Then we repeat this for any other algorithm
a2. NFL tells us that the average performance will be the same
for both algorithms, regardless of which pair of algorithms we selected. Since
it is true for all pairs of algorithms, and since random search is one of these
algorithms, this means that no algorithm is any better (or worse) than a random
search, when averaged over all possible fitness functions. It even means that,
averaged over all possible fitness functions, a hill-descending algorithm will
be just as good as a hill-climbing algorithm at finding high points! (A
hill-descender is like a hill-climber except that it moves to the lowest of the
available points instead of the highest.)
This result seems incredible, but it really is true. The important thing to
remember is the vital phrase "averaged over the set of all possible fitness
functions". The vast majority of fitness functions in that set are totally
chaotic, with the height of any two adjacent points being unrelated. Only a
minuscule number of those fitness functions have the smooth rolling hills and
valleys that we usually associate with a "landscape". In a chaotic landscape,
there are no hills worthy of the name to be climbed. Furthermore, remember that
every point which a hill-climber or descender peeks at counts as having been
"found", even if the algorithm decides not to move there. So if a hill-descender
happens to move adjacent to a very tall spike, the fitness value at that spike
will be recorded and will count in the descender's final performance evaluation.
A landscape picked at random from the set of all possible fitness functions will
almost certainly be just a random mass of spikes (figure 2).
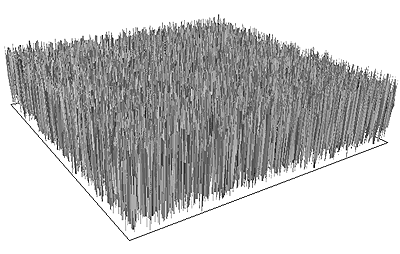
Figure 2. A Random Fitness
"Landscape"
We can already see that the relevance of NFL to real problems is limited. The
fitness landscapes of real problems are not this chaotic. This fact has been
noted by a number of researchers:
In spite of the correctness of this "no-free-lunch theorem"
(Wolpert and Macready 1997) the result is not too interesting. It is easy to
see, that averaging over all different fitness functions does not match the
situation of black-box optimization in practice. It can even be shown that in
more realistic optimization scenarios there can be no such thing as a
no-free-lunch theorem (Droste, Jansen, and Wegener 1999).30
5.4 The Irrelevance of NFL to Dembski's Arguments
NFL is not applicable to biological evolution, because biological evolution
cannot be represented by any algorithm which satisfies the conditions given
above. Unlike simpler evolutionary algorithms, where reproductive success is
determined by a comparison of the innate fitness of different individuals,
reproductive success in nature is determined by all the contingent events
occurring in the lives of the individuals. The fitness function cannot take
these events into account, because they depend on interactions with the rest of
the population and therefore on the characteristics of other organisms which are
also changing under the influence of the algorithm. In other words, the fitness
function of biological organisms changes over time in response to changes in the
population (of the same species and of other species), violating the final
condition listed above. The same also applies to any non-biological simulations
in which the individuals interact with each other, such as the competing
checkers-playing neural nets which are discussed below.
It would do no good to suggest that the interactions between individuals
could be modelled within the optimization algorithm, rather than in the fitness
function. This is prevented by the black-box constraint, which stops the
optimization algorithm having direct access to information about the
environment.
This is similar to the problem of coevolving fitness landscapes raised by
Stuart Kauffman (p. 224-227). Dembski's response to Kauffman, however, does not
address my argument. Nothing that Dembski writes (p. 226) changes the fact that,
in biological evolution, the fitness function at a given time cannot be
determined independently of the state of the population, and therefore NFL does
not apply.31
Moreover, NFL is hardly relevant to Dembski's argument even for the simpler,
non-interactive evolutionary algorithms to which it does apply (those where the
reproductive success of individuals is determined by a comparison of their
innate fitness). NFL tells us that, out of the set of all mathematically
possible fitness functions, there is only a tiny proportion on which
evolutionary algorithms perform as well as they are observed to do in practice.
From this, Dembski argues that it would be incredibly fortuitous for a suitable
fitness function to occur without fine-tuning by a designer. But the alternative
to design is not purely random selection from the set of all mathematically
possible fitness functions. Fitness functions are determined by rules, not
generated randomly. In the real world, these rules are the physical laws of the
Universe. In a computer model, they can be whatever rules the programmer
chooses, but, if the model is a simulation of reality, they will be based to
some degree on real physical laws. Rules inevitably give rise to patterns, so
that patterned fitness functions will be favoured over totally chaotic ones. If
the rules are reasonably regular, we would expect the fitness landscape to be
reasonably smooth. In fact, physical laws generally are regular, in the sense
that they correspond to continuous mathematical functions, like "F = ma", "E =
mc2", etc. With these functions, a small change of input leads to a
small change of output. So, when fitness is determined by a combination of such
laws, it's reasonable to expect that a small movement in the phase space will
generally lead to a reasonably small change in the fitness value, i.e. that the
fitness landscape will be smooth. On the other hand, we expect there to be
exceptions, because chaos theory and catastrophe theory tell us that even smooth
laws can give rise to discontinuities. But real phase spaces have many
dimensions. If movement in some dimensions is blocked by discontinuities, there
may still be smooth contours in other dimensions. While many potential mutations
are catastrophic, many others are not.
Dembski might then argue that this only displaces the problem, and that we
are incredibly lucky that the Universe has regular laws. Certainly, there would
be no life if the Universe did not have reasonably regular laws. But this is
obvious, and is not specifically a consequence of NFL. This argument reduces to
just a variant of the cosmological fine-tuning argument, and a particularly weak
one at that, since the "choice" to have regular laws rather than chaotic ones is
hardly a very "fine" one.
Although it undermines Dembski's argument from NFL, the regularity of laws is
not sufficient to ensure that real-world evolution will produce functional
complexity. Dembski gives one laboratory example where replicating molecules
became simpler (the Spiegelman experiment, p. 209). But it does not follow that
this is always so. Dembski has not established any general rule. I would suggest
that, because the phase space of biological evolution is so massively
multidimensional, we should not be surprised that it has produced enormous
functional complexity.
6. The Uniform-Probability Method
Operator... Give me Information. Song by William Spivery
6.1 Derived Design
Dembski tells us that he has two different arguments for design in nature. As
well as attempting to show that there exist in nature phenomena which Darwinian
evolution does not have the ability to generate (such as the bacterial
flagellum), Dembski also deploys another argument. Even if Darwinian evolution
did have that ability, he argues, it could only have it by virtue of there
having been design involved in the selection of the initial conditions
underlying evolution.
The Darwinist therefore objects that "real life" Darwinian
evolution can in fact generate specified complexity without smuggling it in
after all. The fitness function in biological evolution follows directly from
differential survival and reproduction, and this, according to the Darwinist,
can legitimately be viewed as a "free lunch".... If this objection is
conceded, then the only way to show that the Darwinian mechanism cannot
generate specified complexity is by demonstrating that the gradients of the
fitness function induced by differential survival and reproduction are not
sufficiently smooth for the Darwinian mechanism to drive large-scale
biological evolution. To use another Dawkins metaphor, one must show that
there is no gradual way to ascend "Mount Improbable." This is a separate line
of argument and one that I shall take up in the next chapter [which addresses
irreducible complexity and the bacterial flagellum]. Here, however, I want to
show that this concession need not be granted and that the displacement
problem does indeed undercut Darwinism. [p. 208]
This is an argument for what I will call derived design. (Dembski uses
the term derived intentionality.) It does not argue for design in a
particular event (such as evolution of some structure), but merely argues that
design must have been involved at some point or other in the causal chain of
events leading to some phenomenon that we observe.
We have already seen this argument cast in terms of fine-tuning of fitness
functions. Dembski also casts it in terms of specified complexity.
Earlier, specified complexity was introduced as something to be inferred when we
had eliminated all the natural hypotheses we could think of to explain an event.
But now Dembski is telling us that, even if we cannot eliminate Darwinian
evolution as an explanation, we should still make an inference of derived design
if we observe specified complexity. Clearly, then, this is a different meaning
of specified complexity. This new meaning is an observed property of a
phenomenon, not an inferred property of an event. It indicates that the
phenomenon has a complex (in a special sense) configuration. This property also
goes by the name of complex specified information (CSI).
Note that this is another purely eliminative method; it infers design from
the claimed absence of any natural process capable of generating CSI. If the
claim were true, it could be considered a proscriptive generalization, but we
will see that the claim has no basis whatsoever.
6.2 Complex Specified Information (CSI)
Dembski devises his own measure of how complex a phenomenon's configuration
is, and calls it specified information (which I'll abbreviate to SI). He
calculates this measure by choosing a specification (as described in 3.1 above) and then
calculating the probability of an outcome matching that specification as if
the phenomenon was generated by a process having a uniform probability
distribution. A uniform probability distribution is one in which all
possible outcomes (i.e. configurations) have the same probability, and Dembski
calculates the SI on this basis even if the phenomenon in question is known not
to have been generated by such a process. (This will be considered more
carefully in the next section.) The probability calculated in this way is then
converted into "information" by applying the function I(R) =
-log2P(R), i.e. the information is the negation of the
logarithm (base 2) of the probability, and he refers to the resulting measure as
a number of bits.
If the SI of a phenomenon exceeds a universal complexity bound of 500
bits, then Dembski says that the phenomenon exhibits complex specified
information (or CSI).32 The
universal complexity bound is obtained directly from Dembski's universal
probability bound of 10-150, since
-log2(10-150) is approximately 500. Dembski also refers to
CSI as specified complexity, using the two terms interchangeably. As
noted above, this meaning of specified complexity is different from the one we
encountered earlier. I'll call it uniform-probability specified
complexity. To be clear: eliminative specified complexity is an
inferred attribute of an event, indicating that we believe the event was highly
improbable with respect to all known causal mechanisms; uniform-probability
specified complexity (or CSI) is an observed attribute of a phenomenon,
indicating that the phenomenon has a "complex" configuration, without regard to
how it came into existence. We will see that Dembski's notion of "complexity" is
very different from our normal understanding of the word.
6.3 Evidence For The Uniform-Probability
Interpretation
The fact that the probability used to calculate SI is always based on a
uniform probability distribution is extremely important. Dembski uses a uniform
(or "purely random") distribution even if the phenomenon is known to have been
caused by a process having some other probability distribution. Since this is
not explicitly stated by Dembski, and may seem surprising, I will present
several items of evidence to justify my interpretation.
- Exhibit #1 -- The derived design argument
-
As argued in 6.1 above,
Dembski's derived design argument implies that SI is an observed property of a
phenomenon, which allegedly allows us to infer design in the distant past
regardless of what subsequent natural processes might have led to the
phenomenon. There are therefore no relevant probability distributions under
which to calculate the probability for SI. We must use some default
probability distribution, and a uniform distribution seems to be the only
candidate.
- Exhibit #2 -- The Weasel program26
-
Here Dembski specifically mentions a uniform probability distribution:
For instance, in Dawkins's METHINKS-IT-IS-LIKE-A-WEASEL example
(see section 4.1), the phase space consists of all sequences 28 characters
in length comprising upper case Roman letters and spaces (spaces being
represented by bullets). A uniform probability on this space assigns equal
probability to each of these sequences--the probability value is
approximately 1 in 1040 and signals a highly improbable state of
affairs. It is this improbability that corresponds to the complexity of the
target sequence and which by its explicit identification specifies the
sequence and thus renders it an instance of specified complexity (though as
pointed out in section 4.1, we are being somewhat loose in this example
about the level of complexity required for specified
complexity--technically, the level of complexity should correspond to the
universal probability bound of 1 in 10150). [p.
188-189]
This seems clear. However, Dembski goes on to say that "E [the
evolutionary algorithm] has in fact not generated specified complexity at all
but merely shifted it around" (p. 195). (By this point Dembski has switched to
another version of the Weasel program, but the change is inconsequential.)
Although he fails to state it explicitly, the implication is that the outcome
does exhibit specified complexity, though this was "smuggled" into the
program and not generated by it.
- Exhibit #3 -- Evolutionary algorithms
-
My next piece of evidence comes from Dembski's accounts of Tom Schneider's
binding site simulation33
(pp. 213-218) and the checkers-playing neural nets of Kumar Chellapilla and
David Fogel (pp. 221-223), which I will describe later. These
programs have a high probability of producing a good solution (as has been
confirmed to me by their programmers). Since Dembski asserts that the outcomes
exhibited specified complexity (CSI), which implies a low probability of
producing a specified result (a good solution), it follows that he must have
been estimating the probability with respect to some probability distribution
other than the true one. The only apparent candidate is a uniform probability
distribution.
- Exhibit #4 -- The SETI sequence
-
One of Dembski's examples (pp. 6-9) is an event from the film
Contact, starring Jodie Foster, in which astronomers at SETI (the
Search for Extraterrestrial Intelligence) detect a radio signal of
extraterrestrial origin. The signal comprises a sequence of 1126 beats and
pauses, representing the first 25 prime numbers: 2, 3, 5, 7, ..., 101. Each
prime number is represented by a sequence of beats equal to the number, with
consecutive numbers separated by a pause. Converting the beats to 1s and the
pauses to 0s, the signal can be represented by a sequence of 1126 binary
digits (bits), beginning "110111011111011111110...". The fictional astronomers
immediately recognized this signal as having an intelligent origin.
Dembski tells us that the SETI sequence exhibits specified complexity (p.
359). On pp. 143-144 he gives an abbreviated 1000-bit version of this
sequence, telling us that it has a probability of 1 in 21000,
giving SI of over 500 bits (presumably 1000 bits). The latter example is based
on a known cause (intelligent agency or coin tossing), but presumably the
sequence has the same SI regardless of its cause. After all, we don't know the
true cause of the SETI sequence, yet Dembski still tells us it exhibits
specified complexity. It is very unlikely that the SETI sequence was produced
by the equivalent of coin tossing. A much more likely scenario is that the
extraterrestrials have programmed a computer to generate the sequence
automatically. In that case, we're back to the same sort of situation as the
Weasel program. Furthermore, if we were considering all relevant chance
hypotheses, we should consider the possibility that the two alternative
outcomes of each beat/pause were not equally likely. In the absence of any
other information, the best estimate of the probabilities of beat and pause
would be 1102/1126 and 24/1126 respectively, since we observed 1102 beats and
24 pauses. With these probabilities (and still assuming that each beat/pause
is independent of the others), the probability of receiving the SETI sequence
would be (1102/1126)1102 × (24/1126)24 = 3.78 ×
10-51, considerably larger than the universal probability bound of
10-150. I therefore conclude that Dembski calculates the SI of the
SETI sequence on the basis of the beats and pauses having equal probability
(1/2) and that the sequence exhibits 1126 bits of SI.
- Exhibit #5 -- URF13
-
Finally, I must mention a counterexample to my uniform-probability
interpretation. Dembski considers the case of a gene, T-urf13, which occurs in
a particular strain of maize (pp. 218-219). This gene codes for a protein
product called URF13. In determining whether URF13 exhibits CSI, Dembski
starts by calculating a probability of 2083, on the grounds that
the minimal functional size of URF13 is 83 amino acids and that there are 20
possible amino acids. So he is assuming that URF13 is drawn from a uniform
probability distribution over the space of all possible sequences of 83 amino
acids. He then points out that the probability is really higher than this as
we must allow for the possibility of other sequences having the same function
as URF13, i.e. other sequences matching the same specification. So far, then,
this supports the uniform-probability interpretation. Further down the page,
however, he argues that the probability on which SI must be based is higher
still:
What if any way we sliced it, the improbabilities computed
turned out to be less than the universal probability bound? Would that
demonstrate that CSI had been naturalistically generated? No. First off,
there is no reason to think that non-protein-coding gene segments themselves
are truly random--as noted above, T-urf13, which is composed of such
segments, is homologous to ribosomal RNA. So it is not as though these
segments were produced by sampling an urn filled with loosely mixed nucleic
acids. What's more, it is not clear that recombination itself is truly
random. [p. 219]
Now Dembski is saying that we shouldn't just calculate SI based on a
uniform probability (urn) model, but should take into account the causal
processes which we think are operating. But this contradicts the computer
examples given above, where we knew the actual causal process (execution of a
computer program) and that process gave a specified result with high
probability, yet Dembski told us that the result exhibited CSI
anyway.
Although the evidence is inconclusive, it seems to predominantly favour the
uniform-probability interpretation, and that is the one that I will consider
hereafter. But let me briefly look at the alternatives:
-
SI is based on the probability with respect to the true causal process
responsible for the event. This would make SI useless for the purpose of
making inferences about the cause of a phenomenon. We would need to know the
cause in order to infer the cause! Furthermore, it would be meaningless to say
that a designed phenomenon exhibits CSI, since there is no probability
distribution with respect to which we can calculate the SI of a designed
phenomenon (as Dembski tells us that design is not a probabilistic process).
This interpretation is clearly untenable.
-
SI is based on our best understanding of the causal processes which we
think might underlie the event which gave rise to the observed phenomenon. But
this is just the chance-elimination method again. We calculate the SI under
the best chance hypothesis we can think of (the one conferring the highest
probability on a detachable rejection region). If the SI under this chance
hypothesis is high enough (probability low enough), we reject this chance
hypothesis and infer design. By implication, we have already considered and
rejected all the inferior chance hypotheses that we could think of (those
conferring only lower probabilities on detachable rejection regions). In that
case, the assertion that a phenomenon exhibits CSI is merely an assertion that
it is improbable under all the chance hypotheses we can think of. In other
words, this is the same argument from ignorance which was addressed
earlier.
If Dembski insists that he has only one method of design inference and that
it's the chance-elimination method, then he needs to explain away the exhibits
above and justify his introduction of the terms "complexity" and "information".
The chance-elimination method uses a statistical (probabilistic) technique for
eliminating hypotheses. This has nothing to do with complexity or information.
Transforming probabilities by applying the trivial function I =
-log2P does not magically convert them into complexity or
information measures. It only serves to obfuscate the nature of the
argument.
My guess is that Dembski has failed to notice that he has two different
methods. One reason for his confusion may be that all the chance hypotheses he
ever considers in his examples are ones which give rise to a uniform probability
distribution, with the sole exception of one trivial case (p. 70).
Dembski also seems to consider uniform probability distributions "privileged"
in some sense (p. 50). Referring to the phase space of an optimization
algorithm, he writes:
Moreover, such spaces typically come with a uniform probability
that is adapted to the topology of the phase space. What this means is that
Ω [the phase space] possesses a uniform probability measure U
adapted to the metric on Ω so that geometrically congruent pieces of
Ω get assigned identical probabilities (see section 2.2). [p.
188]
But a phase space (as the search space of an optimization algorithm) does not
come with a probability distribution attached. It is simply a space of possible
solutions which we are interested in searching.34
6.4 The Choice of Phase Space
Although basing SI on a uniform probability distribution helps to make it
independent of the causal process which produced the phenomenon, it cannot make
it completely independent. Given a phase space (or reference class of
possibilities, as Dembski calls it), there is only one possible uniform
distribution, the one in which all outcomes have equal probability. But how do
we choose a phase space? In the case of the SETI sequence it may seem obvious
that the relevant phase space is the space of all possible bit sequences of
length 1126. But why should we assume that the sequence was drawn from a space
of 1126-bit sequences, and not sequences of variable length? Why should we
assume that beat and pause were the only two possible values? The supposed
extraterrestrials could have chosen to transmit beats of varying amplitudes.
Similar problems arise elsewhere. On p. 166, Dembski calculates the
complexity of the word METHINKS as -log2(1/278) = 38
bits.35
This is based on a phase space of strings of 8 characters where each character
has 27 possibilities (26 letters in the alphabet plus a space). He does not
consider the possibility of more or fewer than 8 characters. So clearly Dembski
has a rule that we should only consider possible permutations of the same
number of characters (or components) as we actually observed. No
justification is given for such a rule, and it still leaves us with an arbitrary
choice to make regarding the unit of permutation. In the case of METHINKS, it
might be argued that the only sensible unit of permutation is the character. But
in other cases we have to make a choice. In a sentence, should we consider
permutations of characters or of words? In a genome, should we consider
permutations of genes, codons, base pairs or atoms?
Even more problematical is the range of possible characters. Here Dembski has
chosen the 26 capital letters and a space as the only possibilities. But why not
include lower case letters, numerals, punctuation marks, mathematical symbols,
Greek letters, etc? Just because we didn't observe any of those, that doesn't
mean they weren't real possibilities. And if we are to consider only the values
that we actually observed, why did Dembski include all 26 letters of the
alphabet? Many of those letters were not observed in the word METHINKS or even
in the longer sentence in which it was embedded. Perhaps Dembski is relying on
knowledge of the causal process which gave rise to the word, knowing that the
letters were drawn from a collection of 27 Scrabble tiles, say. But to rely on
knowledge of the causal process makes the criterion useless for those cases
where we don't know the cause. And the whole purpose of the criterion was to
enable us to infer the type of causal process (natural or design) when it is
unknown.
The selection of an appropriate phase space is not such a problem in standard
Shannon information theory,36
because we are concerned there with measuring the information transmitted (or
produced) by a process. Dembski, on the other hand, wants to measure the
information exhibited by a given phenomenon, and from that make an
inference about the causal process which produced the phenomenon. This requires
him to choose a phase space without knowing the causal process, the result being
that his phase spaces are arbitrary.
Dembski devotes a section of his book (pp. 133-137) to the problem of
selecting a phase space, but fails to resolve the problem. He argues that we
should "err on the side of abundance and include as many possibilities as might
plausibly obtain within that context" (p. 136). But this means that we err on
the side of overestimating the amount of information exhibited by a phenomenon,
and so err on the side of falsely inferring design. This is hardly reasonable
for a method which is supposed to infer design reliably. In any case, Dembski
doesn't follow his own advice. We just saw in the METHINKS example that he chose
a space based on only 27 characters--hardly the maximum plausible number given
no knowledge of the causal process.
Given that SI is based on a uniform probability distribution, there is little
doubt that CSI exists in nature. Indeed, all sorts of natural phenomena can be
found to exhibit CSI if a suitable phase space is chosen. Take the example
mentioned earlier of craters on the Moon. If we take the space of all
theoretically possible lunar landscapes and randomly pick a landscape from a
uniform distribution over that space, then the probability of obtaining a
landscape exhibiting such circular formations as we actually observe on the Moon
is extremely small, easily small enough to conclude that the actual lunar
landscape exhibits CSI. Even the orbits of the planets exhibit CSI. If a
planetary orbit were randomly picked from a uniform probability distribution
over the space of all possible ways of tracing a path around the Sun, then the
probability of obtaining such a smooth elliptical path as a planetary orbit is
minuscule.
6.5 The Law of Conservation of Information
The Law of Conservation of Information (hereafter LCI) is Dembski's
formalized statement of his claim that natural causes cannot generate
CSI; they can only shuffle it from one place to another. The LCI states that, if
a set of conditions Y is sufficient to cause X, then the SI exhibited by Y and X
combined cannot exceed the SI of Y alone by more than the universal complexity
bound, i.e.
I(Y&X) <= I(Y) + 500
where I(Y) is the amount of SI exhibited by Y. (I have made Y
antecedent to X, rather than the other way around, for the sake of consistency
with Dembski's explanation on pages 162-163.)
Despite its name, the LCI is not a conservation law. Since Dembski
acknowledges that small amounts of SI (less than 500 bits) can be generated by
chance processes (pp. 155-156), the LCI cannot be construed as a
conservation law in any reasonable sense of the term. It is, rather, a
limit on how much SI can be generated.
My discussion of the LCI below will be based on my uniform-probability
interpretation of SI. However, in case Dembski rejects this interpretation, let
me first consider what the LCI would mean if SI is based on the true
probabilities of events. It would then be just a disguised version of Dembski's
old Law of Small Probabilities, from The Design Inference, which states
that specified events of small probability (less than 10-150) do not
occur. He has merely converted probability to "information" by applying the
function I = -log2P to each side of an inequality, with
the probabilities conditioned on the occurrence of Y. To see this, let X be a
specified event which has occurred as a result of Y. Then, by the Law of Small
Probabilities,
- P(X|Y) >= 10-150
- <=> P(Y&X) >= P(Y) ×
10-150 (since P(Y&X) = P(Y) × P(X|Y))
- <=> I(Y&X) <= I(Y) + 500 (taking
log2 of both sides).
In this case, the LCI is just a probability limit and has nothing to do with
information or complexity in any real sense. I will therefore not consider this
interpretation any further.
6.6 Counterexample: Checkers-Playing Neural Nets
My first counterexample to the LCI is one which Dembski bravely introduces,
namely the evolving checkers-playing neural nets of Chellapilla and Fogel (pp.
221-223).37 I'll
start with a brief description of the evolutionary algorithm. Neural nets were
defined by a set of parameters (the details are unimportant) which determined
their strategy for playing checkers. At the start of the program run, a
population of 15 neural nets was created with random parameters. They had no
special structures corresponding to any principles of checkers strategy. They
were given just the location, number and types of pieces--the same basic
information that a novice player would have on his/her first game. In each
generation, the current population of 15 neural nets spawned 15 offspring, with
random variations on their parameters. The resulting 30 neural nets then played
a tournament, with each neural net playing 5 games as red (moving first) against
randomly selected opponents. The neural nets were awarded +1 point for a win, 0
for a draw and -2 for a loss. Then the 15 neural nets with the highest total
scores went through to the next generation. I'll refer to the (+1, 0, -2)
triplet as the scoring regime, and to the survival of the 15 neural nets
with the highest total score as the survival criterion.
The neural nets produced by this algorithm were very good checkers players,
and Dembski assumes that they exhibited specified complexity (CSI). He gives no
justification for this assumption, but it seems reasonable given the
uniform-probability interpretation. Presumably the specification here is,
broadly speaking, the production of a good checkers player, and the phase space
is the space of all possible values of a neural net's parameters. If the
parameters were drawn randomly, the probability of obtaining a good checkers
player would be extremely low. Since the output of the program exhibited CSI,
Dembski needs to show that there was CSI in the input. To his credit, Dembski
doesn't take the easy way out and claim that the CSI was in the computer or in
the program as a whole. The programming of the neural nets was quite independent
of the evolutionary algorithm. Instead Dembski claims that the CSI was
"inserted" by Chellapilla and Fogel as a consequence of their decision to keep
the "criterion of winning" constant from one generation to the next! But a
constant criterion is the simplest option, not a complex one, and the
idea that such a straightforward decision could have inserted a lot of
information is absurd.
As we saw earlier, the fitness function reflects the problem to be solved. In
this case, the problem is to produce neural nets which will play good checkers
under the prevailing conditions. Since the conditions under which the evolved
neural nets would be playing were (presumably) unknown at the time the algorithm
was programmed, it might be argued that the choice of winning criterion was a
free one. The programmers could therefore have chosen any criterion they liked.
Nevertheless, the natural choice in such a situation is to choose the simplest
option. In choosing a constant winning criterion, that is what the programmers
did. Since they had no reason to think the neural nets would find themselves in
a tournament with variable winning conditions, there was no reason to evolve
them under such conditions.
Contrary to Dembski's assertion that the choice of a constant criterion "is
without a natural analogue", the natural analogue of the constant winning
criterion is the constancy of the laws of physics and logic.
Also, their choice is without a natural analogue. Chellapilla and
Fogel kept constant their criterion for "tournament victory." For biological
systems, the criterion for "tournament victory" will vary considerably
depending on who is playing in the tournament. [p. 223]
It's not clear what Dembski means by "the criterion for tournament victory".
However, the fact that a biological system's success depends on who is "playing
in the tournament" certainly does have an analogue in Chellapilla and Fogel's
algorithm. The success of a neural net was dependent on which other neural nets
were playing in the tournament.
Before considering some further objections to Dembski's claim, I need to
decide what he means by "criterion of winning". Does he mean just the scoring
regime? Or does he mean the entire set of tournament rules: the selection of
opponents, the scoring regime and the survival criterion? For brevity, I will
consider only the scoring regime, but similar arguments can be made in respect
of the other elements of the tournament rules.
Dembski insists that the SI "inserted" by Chellapilla and Fogel's choice is
determined with respect to "the space of all possible combinations of local
fitness functions from which they chose their coordinated set of local fitness
functions". It's not clear what Dembski means by fitness functions here.
As we've seen, in a situation where the success of an individual depends on its
interactions with other individuals in the population (in this case the
population of neural nets), the fitness function varies as the population
varies, since the fitness of an individual is relative to its environment, which
includes the rest of the population. Dembski seems to recognize this, since he
writes:
There is not even a fitness function defined over the entire space
of checker-playing neural nets. Instead, each collection of 30 neural nets
gets its own local fitness function that assigns fitness depending on how a
neural net fares in a tournament with other neural nets... [p. 222]
When Dembski refers to a "local fitness function" here, he apparently means
the fitness function of one particular generation.38 But,
contrary to Dembski's claim, the sequence of fitness functions was not
"coordinated" by Chellapilla and Fogel. It was dependent on the evolution of the
population of neural nets. So it makes no sense to talk about Chellapilla and
Fogel selecting from "the space of all possible combinations of local fitness
functions". It would make some sense, however, to talk about them
selecting from the phase space of all possible time-dependent scoring regimes
(one triplet per generation), and I will assume that this is what Dembski
means.
For the reasons already given, it is hardly reasonable to consider the
scoring regime to have been selected from such a phase space. Nevertheless, even
if we do so, the amount of SI inserted by Chellapilla and Fogel's choice was
minimal. This is because the SI must be based on a rejection region consisting
of all the possible time-dependent scoring regimes which would have performed as
well as Chellapilla and Fogel's or better. Suppose that, instead of using a
constant scoring regime, the program randomly generated a new scoring triplet
(W, D, L) for each generation, subject only to the constraint that W > D >
L. This constraint is not an artificial imposition; it is a characteristic of
the problem to be solved. If the problem was to find good players for suicide
checkers (where the object of the game is to "lose"), the constraint would be L
> D > W. Since SI is based on a uniform probability distribution, the
values of W, D and L would be drawn from a uniform probability distribution over
some continuous range, say [+2, -2]. We are only concerned with the relative
values of W, D and L, so the choice of range is arbitrary and could itself be
made randomly at the start of each run. The question then is how often such a
program would perform as well as the original, i.e. would produce as good
players in the same amount of time. If, for the sake of example, the revised
program performs as well as the original on 1/8 of occasions (out of a
sufficiently large sample), this means that 1 in 8 time-dependent regimes
performs as well as Chellapilla and Fogel's. The SI of Chellapilla and Fogel's
regime would then be only -log2(1/8) = 3 bits. For Dembski to
maintain his claim that this choice inserted CSI, the onus is on him to show
that the proportion of regimes performing as well as Chellapilla and Fogel's is
less than 1 in 10150, and that seems very unlikely to be the
case.
In case Dembski has a problem with even the natural constraint that I
suggested above, let's consider an alternative which has no prior constraints.
Suppose that, at the start of each run, the program draws 6 random numbers from
an arbitrary range (as above). Call these numbers W-, W+,
D-, D+, L-, L+. For each generation,
the program generates a new scoring triplet (W, D, L), selecting these
parameters randomly from uniform probability distributions over the ranges
[W-, W+], [D-, D+] and
[L-, L+] respectively. Many program runs will fail to
produce good checkers players at all (though they may produce players who are
good at playing suicide checkers or good at forcing a draw). However, on a small
proportion of runs (1/720 on average), it will just so happen that W+
> W- > D+ > D- > L+>
L-, and on these runs we can expect the program to produce good
checkers players.39 So, if
we run the program enough times, the output will sometimes exhibit CSI
even though there was no SI in the scoring regime. Alternatively, we can say
that a successful regime like Chellapilla and Fogel's40 has
SI of -log2(1/720) = 9.49 bits, plus a few bits to allow for the fact
that not all successful regimes perform as well as this one, as discussed
above.
Having seen that very little if any SI was "inserted" through the choice of
scoring regime, Dembski might choose to focus on other parameters, such as the
population size. The beauty of Chellapilla and Fogel's algorithm, however, is
that it has very few parameters and even those few could be varied considerably
without adversely affecting the performance of the program. Nothing has been
fine-tuned. Just as in the case of the scoring regime, the selection of those
other parameters therefore involves little SI.
6.7 Counterexample: Mathematical Sequences
As discussed earlier, Dembski apparently considers that the 1126-bit SETI
prime sequence exhibits 1126 bits of SI. But, if this is so, it follows that an
n-bit sequence would exhibit n bits of SI. So a computer program
which outputs this sequence can produce as much SI as we like, simply by letting
the program run for long enough. The SI of the output could run into millions of
bits and easily exceed the SI of the program, no matter how big that program is.
Perhaps the task of generating primes is too intractable for this to be a
practical possibility. In that case we can just pick a simpler sequence, such as
the Fibonacci sequence. If we take a really simple sequence like the
Champernowne sequence (p. 64), we can even program it in just a few machine code
instructions and run it on a bare computer (with no operating system), so the
total SI of the software is less than 500 bits, not even enough to constitute
CSI.
I give the following justification for asserting that the SI of a program is
no greater than the length of the program. Consider a given program of length N
bits. By analogy to Dembski's METHINKS example (p. 166), I argue that I can take
as my phase space the space of all programs of the same length as my given
program. Then the probability of drawing any given program (i.e. sequence of
bits) from a uniform distribution over this space is 1/2N, so the
information of a particular program is -log2(1/2N) = N
bits. The SI exhibited by the program may be less than this (if more than one
program matches the same specification as the given program) but it cannot be
more.
Since the SI of the program is finite (N bits) but the SI of the output
sequence is unlimited, the program can generate an unlimited amount of SI.
Let me address all the objections that Dembski might make to this
argument:
-
"All the SI in the output was contained in the program." This means that
the program contains an unlimited amount of SI. I doubt that Dembski would
like to take the position that a trivial program can contain an unlimited
amount of SI.
-
"The SI of a mathematical sequence is limited to the length of the program
that is needed to generate it." This is true of algorithmic information
(Kolmogorov complexity),41 but
not of Dembski's SI.
-
"The output of a deterministic process does not exhibit SI because it is
not contingent, as required by the Explanatory Filter." But this would
be making the evaluation of SI dependent on the type of causal process leading
to the phenomenon, the very issue that is in question. If the SETI sequence
exhibits CSI when it is received from outer space, why should the same
sequence not exhibit CSI when it is produced by a computer? And for all we
know, the SETI sequence might also have been generated by a deterministic
computer program (programmed by ETs). In any case, we can make our computer
program non-deterministic if required, e.g. by starting the prime sequence
from a randomly selected prime.
The problem for Dembski is that highly patterned phenomena are tightly
specified, giving them low probability and therefore high SI. Unlike algorithmic
information (Kolmogorov complexity), which is a measure of incompressibility, SI
correlates with compressibility. Highly compressible sequences like the
SETI sequence exhibit high SI. Dembski seems quite happy with this fact:
It is CSI that within the Chaitin-Kolmogorov-Solomonoff theory of
algorithmic information identifies the highly compressible, nonrandom strings
of digits... [p. 144]
So Dembski's information (SI) tends to vary inversely with algorithmic
information. A highly compressible sequence can be high in SI but low in
algorithmic information. Dembski leads us to believe that his CSI is equivalent
to the term specified complexity as used by other authors, giving the
following quotation from Paul Davies' book The Fifth Miracle no less than
four times:
Living organisms are mysterious not for their complexity per
se, but for their tightly specified complexity. [p. 180]
Yet, if we read The Fifth Miracle, we find that Davies uses complexity
in the sense of algorithmic information (Kolmogorov complexity), and not
Dembski's probability-under-a-uniform-distribution sense. Davies also calls his
measure specific randomness, whereas Dembski identifies CSI, in the quote
above, with nonrandom strings.42
In a similar vein, Dembski quotes Leslie Orgel:
Living organisms are distinguished by their specified complexity.
Crystals such as granite fail to qualify as living because they lack
complexity; mixtures of random polymers fail to qualify because they lack
specificity. [p. 229n5]
But, by Dembski's definition, crystals have high complexity, because
the probability of obtaining a crystal shape by purely random combination of
molecules is very small. Like Davies, Orgel defines complexity in terms of "the
minimum number of instructions needed to specify the structure".43
So, contrary to Dembski's implications, his concept of specified complexity
is quite different from that of Davies and Orgel.44
6.8 Dembski's Mathematical Justification
Dembski claims to have provided a mathematical justification for his LCI.
Since we've just seen that counterexamples to the LCI can easily be found, there
must be something wrong with the mathematical justification. In fact, the errors
aren't too hard see.
The first 4 pages of Dembski's justification (pp. 151-155) are concerned with
showing that the LCI is true for deterministic processes. The error in this
argument can be seen most clearly by starting from the following equation (p.
152):
I(A&B) = I(A) + I(B|A).
Since B (the result of a deterministic process) is entirely determined by A
(the antecedent conditions), argues Dembski, I(B|A) = 0, and therefore
I(A&B) = I(A). Thus, no new information has been produced by
the process. (I've simplified Dembski's notation a little.)
The equation above was derived from the following basic probability equation,
simply by transforming both sides of the equation by the trivial transformation
I(E) = -log2P(E):
P(A&B) = P(A) × P(B|A)
which Dembski states in the following form (pp. 128-129):
P(B|A) = P(A&B) / P(A).
So Dembski's result is just another way of saying that the result of a
deterministic process occurs with probability 1. But this tells us nothing about
the specified information exhibited by the result, since that is based on a
uniform probability distribution, regardless of the true probability.
For the sake of a concrete example, let's consider one of the mathematical
sequences above, say the 1126-bit SETI prime sequence. Then A is the program
producing the sequence and B is the sequence itself. Then it's true that
P(B|A) (the probability of B given A) is equal to 1, since A always leads
to B. Therefore, applying the transformation I(E) =
-log2P(E), we arrive at I(B|A) = 0. So far, so good.
However, I(B|A) here is not Dembski's specified information, SI. I
assume that the events in question are specified, so the problem has nothing to
do with specification. The problem is that I(B|A) is just P(B|A)
transformed, and P(B|A) is the true conditional probability of the
event, which in this case is 1. SI, on the other hand, is based on the
assumption of a uniform probability distribution, regardless of the true
probability of the event. In the SETI case, the SI exhibited by B is given
by
SI = -log2(P(B|U)) =
-log2(2-1126) = 1126 bits
where U indicates a uniform probability distribution over the space of
all possible 1126-bit sequences.
In short, Dembski is equivocating between two different meanings of
information.
So deterministic processes can generate CSI. However, Dembski could
have avoided this problem by adopting a sensible measure of information, such as
Davies' specified Kolmogorov complexity. With that measure, it would be true
that deterministic processes cannot generate information, since the output of a
deterministic program by definition has the same or less Kolmogorov complexity
than the program. In order to address Dembski's arguments regarding stochastic
processes, I will pretend, for the remainder of this section, that SI cannot be
generated by deterministic processes.
The next stage in Dembski's justification is to argue that purely random
processes cannot generate CSI (pp. 155-157). I will happily agree that this is
so, given the following elaboration of what I mean by it: we consider it
effectively impossible that a highly specified phenomenon (such as a living
organism) could be drawn from a uniform probability distribution over the space
of all possible combinations of the phenomenon's component parts (if we take
sufficiently simple parts). The probability is just too low. This would be what
Richard Dawkins calls single-step selection.45
However, as Dembski points out, purely random processes can produce smaller
amounts of SI, short of CSI, since these correspond to events of larger
probability. In other words, the LCI does not prohibit natural processes from
producing SI.
But what about cumulative selection (to borrow Dawkins' contrasting
term)? Cumulative selection is a series of small steps through the phase space
(the set of all possible configurations), based on trial and error, in which
advantageous steps are retained and used as a basis for further development.
What is Dembski's argument against the generation of CSI by cumulative
selection? In addressing whether a stochastic process can generate CSI (pp.
157-158), Dembski breaks down the process into two stages: a pure chance stage
followed by a deterministic stage. He argues that neither stage can produce CSI,
and so the combined two-stage process cannot do so either. Strangely, Dembski
doesn't mention the possibility of multiple iterations of chance and
deterministic process. But that's exactly what cumulative selection (e.g.
biological evolution) relies on. Each chance stage (random mutation) can produce
a little SI, and natural selection can act to prevent the existing SI being
lost, allowing it to accumulate over time in a ratchet-like process.
Dembski returns to this issue several pages later (pp. 165-166), when he
claims that CSI is holistic, and so cannot be accumulated. But his
argument in support of the claim utterly fails to address the issue. He points
out that the sentence "METHINKS IT IS LIKE A WEASEL" exhibits more SI than the
aggregate set of individual words {A, IS, IT, LIKE, WEASEL, METHINKS}, because
the former consists of a specified sequence of words. Depending on what
specifications we choose, that may be true. But it is irrelevant, as there is
still no limit on the amount of SI that an aggregate can exhibit, given
sufficient words. In any case, Dembski has given us no reason to think that
natural causes are limited to producing randomly ordered aggregates.
The issue is not how much SI a structure can exhibit, but whether that SI can
arise through natural processes, and Dembski gives no reason whatsoever to think
that SI cannot be accumulated over multiple stages of cumulative selection.
Perhaps Dembski would respond that the probability of the whole process
achieving a specified result is the product of the probabilities of the
individual stages, and so will still come out to be below the universal
probability bound. But this would fail to recognize that at each stage there
will be many attempts (many individuals in the population over many
generations), so the probability of success will be far higher than if a single
individual had to jump the hurdle of every stage in succession.
To sum up, Dembski has failed to show that natural processes cannot produce
specified complexity or CSI, however defined. He has simply evaded the issue of
cumulative selection.
Dembski makes the bold claim that his LCI can be considered a "fourth law of
thermodynamics". Since I have shown the LCI to be baseless, I will not consider
this additional claim.
7. The Positive Case For Design
Research! A mere excuse for idleness; it has never
achieved, and will never achieve any results of the slightest
value. Benjamin Jowett (1817-93), classical scholar
and Master of Balliol College, Oxford
Having previously denied the value of comparing hypotheses and relied on a
purely eliminative approach to inferring to design, Dembski changes tack in his
final chapter and attempts to make a positive case for his design hypothesis.
Much of this is based on the premise that specified complexity is a marker of
design, a premise which we have seen to be false. I will ignore those parts, and
concentrate on the additional claims.
7.1 Outline of a Positive Research Program
The Intelligent Design movement has often been criticized for not conducting
or even proposing any research, and Dembski tries to address this concern here
(pp. 311-314). The first part of this "program" is the search for more cases of
specified complexity, i.e. more biological systems for which there is allegedly
no known natural explanation. Beyond this, all he presents is a list of
questions, of which the following is typical:
Construction Problem--How was the designed object constructed?
Given enough information about the causal history of an object, this question
may admit an answer. [p. 313]46
No serious attempt is made to suggest how these questions might be answered.
A list of questions does not constitute a research program!
Dembski's final question is: "Who or what is the designer?", but he quickly
adds that this is not a question of science. How strange. I thought that
Dembski, like other Intelligent Design proponents, was against the drawing of
arbitrary lines between what can and cannot be included in science. Why should
the identity of the designer be a forbidden topic?
7.2 Testability
The design hypothesis has often been criticized for being untestable. What
exactly is meant by testability is unclear, but Dembski uses the term as
a general heading under which to consider a number of more specific
criteria.
- Falsifiability
-
Both supporters and opponents of Intelligent Design have an unfortunate
tendency to assert that "Intelligent Design is/isn't falsifiable", without
clarifying just what the hypothesis is that they are referring to. In fact, as
we've already seen, Dembski's design hypothesis tells us no more than that a
designer was involved somewhere in the causal chain of events leading to the
bacterial flagellum (and possibly other biological systems). The design
hypothesis says nothing at all about the identity, capabilities or aims of the
designer; whether there were many designers or only one; or how and when the
design was implemented.
The philosopher of science Karl Popper maintained that falsifiability is a
necessary condition for a proposition to be considered a scientific
hypothesis, and this principle has often been used as a demarcation criterion
for separating science from pseudoscience. If there is no evidence that could
possibly contradict a hypothesis, then the hypothesis can be maintained
regardless of the evidence. The principle has been weakened by the observation
(attributed to Duhem and Quine) that any hypothesis can be saved from
falsification by abandoning an auxiliary hypothesis. For example, it is
sometimes argued that the finding of a fossilized human skull in a Precambrian
stratum would falsify the theory of evolution. But, even in the face of such
an observation, the theory of evolution could be saved by supposing that there
is some unknown means by which a fossil could sink through old strata.
Nevertheless, we can think in terms of degrees of falsifiability. The theory
of evolution is relatively falsifiable, because there are many
hypothetical observations, like a skull in the Precambrian, which would cause
significant consternation among scientists, requiring the re-evaluation of at
least one well-supported scientific hypothesis.
On the other hand, there is no potential observation which could cause any
such problem for proponents of the design hypothesis, since any observation
whatsoever is consistent with that hypothesis, with or without any auxiliary
hypotheses. Despite this, Dembski claims that the design hypothesis is
falsifiable, while conceding that he has been "fast and loose" in his use of
the term (p. 357). His usage is indicated by the following passage:
If it could be shown that biological systems like the bacterial
flagellum that are wonderfully complex, elegant and integrated could have
been formed by a gradual Darwinian process (which by definition is
non-telic), then intelligent design would be falsified on the general
grounds that one does not invoke intelligent causes when purely natural
causes will do. In that case Occam's razor finishes off intelligent design
nicely. [p.357]
All Dembski is saying here is that the design hypothesis has the potential
to be replaced by a better one. But that's true of any hypothesis, so the
point is quite uninteresting. This is certainly not what Popper meant by
falsifiability.
- Parsimony
-
It is refreshing that, by his invocation of Occam's razor, Dembski seems to
have recognized that the hypothesis of natural evolution is more parsimonious
than the design hypothesis. One is bound to ask, then, why he thinks a
hypothesis (design) which is entirely lacking in detail should be preferred to
a more parsimonious hypothesis (natural evolution) with limited detail.
Clearly design hypotheses have a very privileged status in Dembski's
system.
- Predictive power
-
Predictive power (or predictability as Dembski calls it) is closely
related to falsifiability (in Popper's sense), since a hypothesis is falsified
by making an observation which contradicts a prediction of the hypothesis. For
a hypothesis to make predictions, it must be able to distinguish between those
observations which are possible under the hypothesis and those which are not,
or else those which are more likely than others. The design hypothesis fails
absolutely in this. With no knowledge of the designer's abilities and aims,
all conceivable possibilities appear equally likely.
In an earlier article, Dembski acknowledged the lack of predictive power of
the design hypothesis: "Yes, intelligent design concedes predictability."47
In No Free Lunch he backs away from this concession, offering two
examples of predictions made by the design hypothesis, but neither of these
stands up to scrutiny.
-
First he tells us that "Intelligent design offers us one obvious
prediction, namely, that nature should be chock-full of specified complexity
and therefore should contain numerous pointers to design" (p. 362). Not at
all. The design hypothesis tells us nothing about how many structures were
designed, and Dembski himself previously told us (p. 24) that design does
not necessarily leave any detectable traces. He even writes:
Please note that I am not offering a theory about the
frequency or intermittency with which an unembodied designer imparts
information into the world. I would not be surprised if most of the
information imparted by such a designer will elude us, not conforming to
any patterns that might enable us to detect this designer... [pp.
346-347]
-
Next he appeals to patterns that have been recognized in the evolution of
human technology, arguing that the design hypothesis predicts we should see
such patterns in biological evolution too (p. 362). But why should that be?
Is Dembski now telling us that his unembodied designer is constrained to
follow similar patterns of development to human designers? If so, then that
is an addition to the design hypothesis which does indeed have some
predictive implications. But it cuts both ways. If we find patterns in
evolution which are not typical of human technological development, that
will count as evidence against the design hypothesis. And we do find such
patterns. For example, we see that biological evolutionary changes occur in
piecemeal fashion, with systems being adapted to completely new functions,
where a human designer would redesign the system from the ground up. Often
this leads to suboptimal systems such as the inverted mammalian retina, with
its blind spot, which could be improved by a simple change, easily made by a
human engineer but possibly inaccessible to natural evolution.48 So
Dembski has a choice here: no predictive power or predictive failure. Which
will it be?
Having failed to establish any predictive power for his own hypothesis, he
tries to turn the tables on evolutionary theory, pointing out that
evolutionary theory does not predict such facts as the existence of particular
species (p. 361). This is true, as such facts are the result of unpredictable
historical contingencies. But evolutionary theory certainly does make many
predictions all the same.49
For example, evolutionary theory predicts that there will be a high degree of
congruence between phylogenetic trees derived from morphological studies and
from independent molecular studies. This prediction has been confirmed, and
continues to be confirmed as more species are tested.50
Proponents of Intelligent Design will sometimes argue that much of the
predictive power of evolutionary theory can be assimilated by Intelligent
Design. For example, those who accept the common ancestry of species may argue
that the congruence of phylogenetic trees is a prediction of common ancestry,
regardless of whether the descent from a common ancestor was controlled by a
designer or not. Dembski does not appear to make such an argument, and indeed
is quite ambivalent about common ancestry (pp. 314-316). In any case, the
introduction of a designer into the evolutionary process weakens the
predictions from common ancestry, since it forces us to allow, for example,
for the designer suddenly introducing entirely new genetic material. The only
way to fully preserve the predictive power of evolutionary theory while
introducing a designer is to hypothesize that the designer perfectly mimics
the empirical effects of natural evolution. Such a hypothesis is entirely
superfluous from a scientific point of view.
- Explanatory power
-
The term explanatory power is widely used but difficult to define. I
will not attempt a definition, but will note that, in part, it is another face
of predictive power, referring to the ability to "retrodict" past
observations. In this sense, the design hypothesis is as totally lacking in
explanatory power as in predictive power.
Dembski claims that there are "things that might occur in biology for which
a design-theoretic framework could give a better, more accurate account than a
purely Darwinian and therefore nonteleological framework" (p. 363). To support
this claim, he gives just one example:
To see this, suppose that I were a supergenius molecular
biologist and that I invented some hitherto unknown molecular machine, far
more complicated and marvelous than the bacterial flagellum. Suppose further
I inserted this machine into a bacterium, set this genetically modified
organism free, allowed it to reproduce in the wild, and destroyed all
evidence of my having created the molecular machine. Suppose, for instance,
the machine is a stinger that injects other bacteria and explodes them by
rapidly pumping them up with some gas (I am not familiar with any such
molecular machine in the wild), thereby allowing bacteria endowed with my
invention to consume their unfortunate prey.
Now let us ask the question, If a Darwinist came upon this
bacterium with the novel molecular machine in the wild, would that machine
be attributed to design or to natural selection?" [p. 364]
Dembski tells us that "intelligent design, by focusing on the stinger's
specified complexity, would confirm the stinger's design whereas Darwinism
never could" (p. 364). What nonsense! There is no reason why a Darwinist (i.e.
mainstream scientist) should not consider the possibility of human design,
just as he or she might suspect genetic engineering of an unusual new strain
of tomato, or selective breeding of a dog. In the case of Dembski's stinger,
we would consider such factors as whether the device was within the reach of
current technology, what motive someone might have for creating it, whether
the device had the appearance of an evolved structure, whether there were any
known similar organisms from which this one might have evolved, etc.
In any case, this has nothing to do with whether there is any explanatory
power to the hypothesis that an unknown being designed the bacterial
flagellum. In Dembski's example we would be considering a possible human
designer with known (to some degree) capabilities and motivations.
So evolutionary theory wins out over the design hypothesis in terms of
productivity as a research program, falsifiability, parsimony, predictive power
and (at least by the definition considered above) explanatory power.
7.3 Unembodied Designers
Dembski makes no attempt to conceal the fact that the designer he has in mind
is an unembodied one. Why, he asks, are scientists willing to consider design
involving alien beings (as in SETI) but not unembodied designers? He answers the
question himself:
At least with extraterrestrial intelligences we can guess what
might have happened. But we do not have any experience with unembodied
designers, and that is clearly what we are dealing with when it comes to
design in biology. [p. 359]
This is exactly the point. In the case of SETI, we can consider the
possibility that aliens evolved naturally, developed the technology to send a
radio signal across interstellar space, and decided it was worth trying to
communicate with us. There are enormous uncertainties involved, but we can make
an informed guess about the relative plausibility of such a hypothesis as
compared with the hypothesis that an extraterrestrial radio signal is of natural
origin.
An unembodied designer, on the other hand, is quite another matter. Dembski
defines an unembodied designer as "an intelligence whose mode of operation
cannot be confined to a physical entity located within spacetime" (pp. 333-334).
We have no idea how such a being could exist or even what it means for such a
being to exist. I for one do not rule unembodied beings out of science as a
matter of principle, but it would require some very significant new developments
in science before such a hypothesis could be seriously entertained. In the
meantime, unembodied designers are a highly unparsimonious explanation.
Not only does Dembski fail to explain the nature of his unembodied designer,
he even tries to rule questions about its origin out of bounds:
The who-designed-the-designer question invites a regress that is
readily declined. The reason this regress can be declined is because such a
regress arises whenever scientists introduce a novel theoretical entity. For
instance, when Ludwig Boltzman introduced his kinetic theory of heat back in
the late 1800s and invoked the motion of unobservable particles (what we now
call atoms and molecules) to explain heat, one might just as well have argued
that such unobservable particles do not explain anything because they
themselves need to be explained. [p. 354]
Of course it is true that scientific explanations often create new unanswered
questions. But, in assessing the value of an explanation, these questions are
not irrelevant. They must be balanced against the improvements in our
understanding which the explanation provides. Invoking an unexplained being to
explain the origin of other beings (ourselves) is little more than
question-begging. The new question raised by the explanation is as problematic
as the question which the explanation purports to answer. As Dawkins puts it
(quoted by Dembski):
You have to say something like "God was always there", and if you
allow yourself that kind of lazy way out, you might as well just say "DNA was
always there", or "Life was always there", and be done with it. [p.
353]
Dembski's response is to attack "Dawkins's reductionist view of science" (p.
353). But this is not reductionism. It is the principle that scientific
explanations should actually explain things, and not just beg the
question!
Jay Richards, another proponent of Intelligent Design, also weighs in with
some empty rhetoric: "If a detective explains a death as the result of a murder
by, say, Jeffrey Dahmer, no one says, 'OK, then who made Jeffrey Dahmer?'" (p.
355). Since we know perfectly well that Jeffrey Dahmer existed, there is
obviously no reason for us to ask that question. If, on the other hand, the
detective announced that the victim was killed by an android (a type of
artificial person described by many science fiction novels), and if we had no
independent evidence of the existence of androids, we would certainly demand an
explanation of how such a being could have come into existence.
One thing that Dembski is willing to say about his proposed unembodied
designer is that it probably intervenes in the world through manipulation of
non-deterministic quantum events:
Thermodynamic limitations do apply if we are dealing with embodied
designers who need to output energy to transmit information. But unembodied
designers who co-opt random processes and induce them to exhibit specified
complexity are not required to expend any energy. For them the problem of
"moving the particles" simply does not arise. Indeed, they are utterly free
from the charge of counterfactual substitution, in which natural laws dictate
that particles would have to move one way but ended up moving another because
an unembodied designer intervened. Indeterminism means that an unembodied
designer can substantively affect the structure of the physical world by
imparting information without imparting energy. [p. 341]
For now, however, quantum theory is probably the best place to
locate indeterminism. [p. 336]
No suggestion is made as to how the desired quantum events might be
"induced".
8. Dembski and Peer Review
Empty vessels make the most noise. Traditional proverb
It is usual for academics, particularly those in technical fields, to submit
their work to review by their peers, their fellow academics with expertise in
the relevant fields. This usually takes the form of submission to specialist
journals in which papers must undergo a process of peer review before
publication. In the long run what matters is whether an idea is accepted by the
experts in the relevant field, but the process of peer review provides a first
sifting to weed out half-baked ideas. Academics also present their work to their
peers at academic conferences. However, Dembski, like other Intelligent Design
proponents, eschews these processes, preferring to sell his ideas directly to
the public, and being careful to avoid review by the experts. He is quoted as
having said:
I've just gotten kind of blasé about submitting things to journals
where you often wait two years to get things into print. And I find I can
actually get the turnaround faster by writing a book and getting the ideas
expressed there. My books sell well. I get a royalty. And the material gets
read more.51
Dembski claims to have provided a rational foundation for the Fisherian
approach to statistics and to have discovered a new Law of Conservation of
Information. If these claims were true, they would be of profound importance to
statisticians and information theorists. He has even be hailed by one of his
allies in the Discovery Institute as "the Isaac Newton of information theory".52 Yet
his work on these subjects has not appeared in any journal of statistics or
information theory, and, as far as I can determine, not one professional
statistician or information theorist has approved of this work. Had any done so,
I am sure we would have heard about it from Dembski himself, since he makes a
habit of using informal references as a substitute for peer review:
Mathematicians and statisticians have been far more receptive
[than philosophers] to my codification of design inferences. Take, for
instance, the positive notice of The Design Inference in the May 1999
issue of American Mathematical Monthly as well as mathematician Keith
Devlin's appreciative remarks about my work in his July/August 2000 article
for The Sciences titled "Snake Eyes in the Garden of Eden": "Dembski's
theory has made an important contribution to the understanding of
randomness--if only by highlighting how hard it can be to differentiate the
fingerprints of design from the whorls of chance."53 [p.
372n2]
Keith Devlin is a respected and widely published mathematician, but he is not
a statistician. His article was of a general nature in a popular magazine, not a
scholarly journal, and did not address the details of Dembski's work. The
content of the article overall was rather more negative towards Dembski's work
than the concluding remark suggests.54 The
"positive notice" in the American Mathematical Monthly reads as follows,
in its entirety:
Probability, S, P, L. The Design Inference: Eliminating Chance
Through Small Probabilities. William A. Dembski. Stud. in Prob.,
Induction, & Decision Theory. Cambridge Univ Pr, 1998, xvii + 243 pp,
$54.95. [ISBN 0-521-62387-1] Not a text but a philosophical tract about when
one can infer design behind events of very small probability. Thought
provoking, fun to read, full of interesting examples. SN55
The fact that Dembski has to resort to such barely favourable references for
support indicates the complete lack of acceptance of his work by the experts in
the relevant technical fields.
We are told (by Dembski and the publisher) that The Design Inference
did undergo a review process, though no details of that process are available.
It is interesting to note, however, that The Design Inference originally
constituted Dembski's thesis for his doctorate in philosophy, and that
his doctoral supervisors were philosophers, not statisticians. The publisher
(Cambridge University Press) catalogues the book under "Philosophy of Science".
One suspects that the reviewers who considered the book on behalf of the
publisher were philosophers who may not have had the necessary statistical
background to see through Dembski's obfuscatory mathematics. In any case, much
of the material in No Free Lunch, including the application of Dembski's
methods to biology, did not appear in The Design Inference, and so has
received no review at all.
9. Conclusion
Better is the end of a thing than its
beginning... Ecclesiastes 7:8.
No Free Lunch is characterized by muddled thinking, fallacious
arguments, errors, equivocation and misleading use of technical jargon. Once
these are cleared up, the following conclusions become apparent:
-
The chance-elimination method is nothing but a god-of-the-gaps-argument. It
merely tells us to infer design when we have rejected all the non-design
hypotheses we can think of.
-
In applying the chance-elimination method to a biochemical system (the
bacterial flagellum), Dembski has failed to consider evolutionary explanations
involving change of function. Instead, he merely considers and rejects an
absurd hypothesis based on purely random combination of parts--the tornado in
a junkyard scenario.
-
The No Free Lunch theorems have no applicability to biological
evolution.
-
The argument from alleged fine-tuning of fitness functions turns out to be
just a trivial variation on the well-known argument from cosmological and
terrestrial fine-tuning.
-
Dembski's idiosyncratic concepts of complexity and
information are misleading, and his so-called Law of Conservation of
Information is fatally flawed.
-
Specified complexity (CSI) is not a marker of intelligent design. If
specified complexity is determined according to the uniform-probability
interpretation, then natural processes are perfectly capable of generating it.
If it is determined by the chance-elimination method, then specified
complexity is just a disguise for the god-of-the-gaps argument.
-
Dembski's claims about statistics, information theory, evolutionary
algorithms and thermodynamics have not undergone peer review and have not been
accepted by the experts in those fields.
In short, No Free Lunch is completely worthless, except as a work of
pseudoscientific rhetoric aimed at a mathematically unsophisticated audience
which may mistake its mathematical mumbo jumbo for genuine erudition. However,
since I have been urged to find something positive to write about it, I am
pleased to be able to report that the book has an excellent index.
I am grateful for the assistance of Wesley Elsberry, Jeffrey Shallit, Erik
Tellgren and others who have shared their ideas with me.
Dembski's statistical method for rejecting individual chance hypotheses is
derived from the approach to statistical inference developed by the statistician
and geneticist R. A. Fisher. Although in widespread use due to its intuitive
appeal, this approach is increasingly rejected by statisticians, including those
who advocate the Neyman-Pearson, Bayesian and likelihood approaches. It is not
my intention here to try to settle this long-standing controversy in statistical
theory, but to point out problems with those of Dembski's claims which are
additional to the standard account of Fisher's approach.
Dembski ambitiously claims to have provided a firm rational foundation for
Fisher's statistical approach--something which has always been absent in the
past--enabling him to extend the approach to a wider class of rejection regions
(specifications) than those allowed by Fisher himself, and to provide
non-arbitrary small probability bounds (pp. 45-47). Most of this appendix will
be concerned with showing the major problems inherent in Dembski's methods for
establishing specifications and probability bounds. I will finish by returning
to the subject of whether he has provided a rational foundation for Fisher's
approach.
He also tries to use his extension of Fisher's approach to justify his
eliminative method of inferring design. But this is a red herring. As shown in
section 3.3
above, Dembski's chance-elimination method simply involves applying his
statistical method to each available chance hypothesis in turn, and inferring
design if the statistical method rejects all the chance hypotheses we can think
of. Therefore, in this appendix, I will have nothing further to say about
"sweeping the field of chance hypotheses", as Dembski puts it (p. 67), but will
simply consider Dembski's statistical method in terms of testing an individual
chance hypothesis.
A.1 Specifications
An excellent comparison of the various approaches to statistics can be found
in Howson and Urbach's Scientific Reasoning: The Bayesian Approach.56
This book is strongly recommended to any reader who wishes to understand the
issue in detail. The clarity of exposition makes a refreshing antidote to
Dembski's muddled thinking. Howson and Urbach make two major objections to
Fisher's approach: the lack of a rational foundation, and the dependence of the
outcome on the choice of test-statistic. Here is how they summarize the second
objection:
Fisher's theory as so far expounded is logically inconsistent. The
problem arises because there are always many random variables that may be
defined on any given outcome space, and not all of them lead to the same
conclusion when put to use as the test-statistic in a significance test.
Hence, one test-statistic may instruct you to reject some hypothesis when
another tells you not to."57
This problem is seen most clearly in the potential for tailoring the choice
of test-statistic to the particular outcome observed, guaranteeing that the
rejection region will be narrowly focused on that outcome and that the
calculated probability will be small. For example, in the Caputo case, we might
choose as our test-statistic the indicator function 1E which
maps the observed outcome E to a value of 1 and all other outcomes to a value of
0. This leads to a rejection region consisting of E alone, with the result that
P(R|H) = P(E|H) = (1/2)41. Dembski warns
us about tailoring:
What needs to be precluded, then, is the tailoring of f [the
test-statistic] to E. Alternatively, f needs to be independent (in some
appropriate sense) of the sample E. [p. 54]
Fisher did not allow unlimited tailoring of the test-statistic to the
observed outcome E, but no clear boundary was ever drawn between what was and
was not allowed. He would have allowed the test-statistic used by Dembski in the
Caputo case (the number of Ds in a sequence), but he would not have allowed the
test-statistic just discussed (the indicator function 1E). Neither, I
think, would he have allowed the test-statistic chosen by Dembski in the
Champernowne case, which is discussed below.
In an attempt to resolve this problem, while widening the range of
permissible test-statistics, Dembski introduces some rules which purportedly
constrain us to choose only appropriate test-statistics, and hence only
appropriate rejection regions. Patterns describing the appropriate (or
detachable) rejection regions are called specifications while
those describing inappropriate ones are called fabrications:
Patterns thus divide into two types, those that in the presence of
complexity [low probability] warrant a design inference and those that despite
the presence of complexity do not warrant a design inference. The first type
of pattern I call a specification, the second a fabrication.
Specifications are the non-ad hoc patterns that can legitimately be
used to eliminate chance and warrant a design inference. In contrast,
fabrications are the ad hoc patterns that cannot legitimately be used
to warrant a design inference. As we shall see in chapter 2, the distinction
between specifications and fabrications can be made with full statistical
rigor. [p. 12]
The hollowness of this claim to rigour will soon become apparent.
Let us start by noting that Dembski uses the term rejection function
instead of test-statistic. In fact, he uses the term ambiguously to refer
both to the test-statistic itself and to its probability density function (or
its probability function in the case of a discrete random variable) (pp. 50,
62). I will use the term in the former sense, i.e. as a synonym for
test-statistic.
To illustrate the role of the rejection function in determining the
specification, I will consider another one of Dembski's examples. A sequence of
100 heads and tails is generated, allegedly by tossing a fair coin 100 times,
and a particular pattern is observed in the sequence (pp. 15-18). The first 50
results in the sequence (labelled E) are as follows:
E:THTTTHHTHHTTTTTHTHTTHHHTTHTHHHTHHHTTTTTTTHTTHTTTHH...
On converting to binary digits, the sequence is seen to consist of all the
1-digit binary numbers in ascending order, followed by the 2-digit binary
numbers, and so on:
D: 0|1|00|01|10|11|000|001|010|011|100|101|110|111|0000|0001|0010|0011|...
This sequence is known as the Champernowne sequence (p. 64), and will be
called D.
The first step in choosing a specification is to select what Dembski calls
background knowledge (K). This is, broadly speaking, any subset of
all the knowledge that was available to us before observing the event in
question. In this case, Dembski selects "our knowledge of binary arithmetic and
lexicographic orderings". From this he derives a rejection function, in this
case the indicator function 1D, which maps outcome D to the value 1
and all other outcomes to the value 0. Finally, from this rejection function
Dembski obtains the specification consisting of the single outcome E.
So there are three steps to reaching a specification: select background
knowledge; from background knowledge to rejection function; from rejection
function to specification. The last of these is not entirely unproblematic, but
I will not consider it here. I will just consider the problems of the first two
steps.
-
First, we have enormous latitude in our choice of K. Dembski imposes
what he calls a conditional independence criterion, the effect of which
is to prevent us including any information which directly tells us anything
about the outcome. However, it does not prevent us from including information
the choice of which tells us something about the outcome. This means
that, when we choose K after observing the outcome, our choice of
specification will not be statistically independent of the outcome, and this
is indeed the case in Dembski's own examples.
Consider the example above. Dembski states that K is conditionally
independent (i.e. statistically independent given the chance hypothesis in
question) of the outcome because our knowledge of binary arithmetic and
lexicographic orderings does not tell us anything about whether the sequence E
has occurred. Perhaps so. But our decision to select "our knowledge of binary
arithmetic and lexicographic orderings" as our background information was very
much dependent on the outcome. If, for example, we had observed a sequence of
prime numbers in the coin tosses, we would have selected "our knowledge of
prime numbers and lexicographic orderings" as our background knowledge. Thus,
the rejection function derived from this background knowledge is not
statistically independent of the outcome, and neither is the resulting
specification.
Indeed, Dembski does not claim that his specifications are statistically
independent of the outcome, only that they are independent in "in a certain
well-defined sense" (p. 15). But this is a peculiar sense which is quite
different from both our everyday sense of the word and the technical sense
used by statisticians. Nor is it even well-defined, as we see below.
-
Second, Dembski does not tell us how to derive the rejection function from
our chosen background knowledge. He tells us we must select background
knowledge "that explicitly and univocally identifies the rejection function f"
(p. 72), but this is clearly not the case in the two examples which he gives
us.
Consider again the example above. The selected background knowledge ("our
knowledge of binary arithmetic and lexicographic orderings") clearly does not
univocally identify the rejection function 1D, which is based on
the Champernowne sequence (D). There are many other patterns involving binary
arithmetic and lexicographic orderings on which we could have based our
rejection function, e.g. a simple sequence of consecutive binary numbers:
0|1|10|11|100|101|110|111|1000|1001|1010|1011|1100|1101|1110|1111|...
Dembski's failure to follow his own rule is indicative of the more general
problem. The concept of "deriving" a rejection function or pattern from our
knowledge of binary arithmetic and lexicographic orderings (or any other set
of knowledge) is not well-defined. This vagueness leaves us with further
latitude to tailor the rejection function to the observed outcome.
In case Dembski responds that he could have selected "our knowledge of the
Champernowne sequence" as his background knowledge instead of "our knowledge
of binary arithmetic and lexicographic orderings", let me point out that this
still would not univocally identify a rejection function. We can invent any
number of variants on the Champernowne sequence. If we had observed a
Champernowne sequence preceded by 5 superfluous digits, Dembski could have
chosen the rejection function which counts the number of digits preceding the
Champernowne sequence (or 100 if there is no Champernowne sequence). This
would lead to the specification "Champernowne sequence preceded by 5 or fewer
superfluous digits". If we had observed a variant Champernowne sequence which
excludes all binary numbers with an even numbers of digits, Dembski could have
chosen some other rejection function tailored to this particular variant. And
so on.
The two problems just described combine to allow a high degree of tailoring
of the rejection function (and hence the specification). To see the sort of
extremes to which tailoring could potentially be taken, consider the following
examples.
-
Let us suppose that the judge in the Caputo trial was a proponent of
Dembski's method. Instead of adopting the specification "40 or more Ds", he
might have adopted the narrower specification "22 Ds, then an R, then 18 more
Ds" (based on the single observed outcome E), justifying this choice with the
following argument: "While I was on my school's football team, we lost the
first 22 matches we played, won 1, and then lost the remaining 18. So 22-1-18
is a pre-existing pattern, and I select it as my background knowledge
K. From this, I derive the rejection function 1E, and hence
the specification '22 Ds, then an R, then 18 more Ds'."
It might be argued that the judge's football record is irrelevant to the
Caputo case. But no such relevancy criterion is currently included in
Dembski's method, and, if background knowledge were to be excluded on the
grounds of relevancy, this would be a highly subjective criterion. If we
observe a suspiciously regular pattern with no knowledge of its cause, and
want to test whether it arose from a certain probability distribution, how can
we decide whether any given piece of background knowledge is relevant to
it?
-
Now suppose that the judge had not seen such a sequence before. He might
still argue for the specification "22 Ds, then an R, then 18 more Ds" on the
following grounds: "I haven't seen the sequence 22-1-18 before, but I have
seen the numbers 22, 1 and 18 separately. So I select them to form part of my
background knowledge K, and then derive the rejection function from
them." Since Dembski does not tell us how to derive a rejection function from
a set of background knowledge, he has no grounds for declaring this derivation
illegitimate.
I suspect Dembski's failure to notice problems like these is a result of the
fact that all his examples are extremely simple ones. As soon as one looks at
more complicated examples, the flaws in the method become much more apparent.
Here is a particularly problematic example, from another source:
Suppose ten dice are rolled and we count the sum of them that
comes up. It will be a number from 10 through 60. I want to maintain that
whatever number it turns out to be, that number will not only be unlikely to
have come up but it will have at least one unique and interesting property,
not possessed by any of the other fifty numbers. For example, if it is, say,
25, then that number would be the only perfect square which is itself the sum
of two squares (9 & 16) and is also the only odd number that is the square
of its last digit. The number 27 is the only perfect cube of all of them. The
number 28 is the only one that is the sum of all its divisors smaller than
itself (1, 2, 4, 7, 14). The number 30 is the largest number X such that all
numbers smaller than X with no divisor in common with X (other than 1) are
themselves prime numbers. The number 32 is the smallest power of 2 such that
the next number after it is not a prime (since the next number after 16 is a
prime). The number 36 is the only one that is the product of two squares (4
& 9) and is the only even number that is the square of its last digit. The
number 11 is the smallest palindromic number and 55 is the largest. The number
59 is the largest prime number. And 60 is that number which can be factored in
more ways than any smaller number. I claim that every number from 10 through
60 has at least one unique and interesting property, especially if
non-mathematical properties are included (such as 26 = the number of letters
in the English alphabet; 29 = the number of days during February in a leap
year; 31 = the most points that can be scored in a hand of cribbage, and so
on). In light of this fact, no matter what number comes up as the sum of the
ten dice, we could say, "How amazing: not only is that number highly unlikely
to have come up but it is the only number such that ..." and proceed to
specify the interesting property or properties uniquely possessed by that
number. Then we could ask: "What is the explanation for the fact that that
number came up rather than some other number?" The correct answer is that it
is just a coincidence (or brute fact) that that number came up, and whatever
number had come up, it would have been unlikely to have done so and, further,
there would have been some interesting property or properties possessed only
by that number.58
I suggest that, in this example, no matter what outcome we observed,
Dembski's approach could be used to justify a rejection region consisting of
just the specific observed outcome.
To sum up this section: we now see that Dembski's method for distinguishing
between specifications and fabrications is far from exhibiting the "full
statistical rigor" that he claimed. On the contrary, the distinction between
specifications and fabrications is highly arbitrary, allowing a high degree of
tailoring of the specification to the observed outcome.
A.2 Specificational Resources
But does this matter? In addition to the concept of specifications, Dembski
has also introduced the concept of specificational resources. Although he
doesn't state this explicitly, the purpose of specificational resources is to
compensate for the tailoring of specifications.
Having chosen a specification and calculated the probability of any outcome
matching that specification, Dembski's method requires us to compare this
probability with a probability bound α, and to reject the chance hypothesis
H if P(R|H) < α. In order to establish a value for α,
Dembski introduces the concept of probabilistic resources:
Probabilistic resources comprise the relevant ways an event can
occur (replicational resources) and be specified (specificational resources).
The important question therefore is not What is the probability of the event
in question? but rather What does its probability become after all the
relevant probabilistic resources have been factored in? [p. 21]
The number of probabilistic resources is the product of the number of
replicational resources (ReplRes) and the number of specificational
resources (SpecRes). α is then determined by dividing the number 1/2 by
this product:
α = ½ ÷ (ReplRes × SpecRes).
However, as the last quote suggests, Dembski often writes as if the
probabilistic resources are factored into P(R|H) (by
multiplication) rather than into α (by division). This makes no difference to
the truth value of the inequality P(R|H) < α, of course, but it
seems natural to think in terms of multiplying P(R|H) by
SpecRes as a compensation for the excessive lowering of this probability
which results from narrowly tailoring the specification to the observed
outcome.
SpecRes is the number of potential specifications which we
might have chosen before we were aware of the outcome. However, we only
count potential specifications which have lower (or equal) probability and
"complexity" than our chosen specification (corresponding to our rejection
region R) and we ignore specifications which are subsets of other specifications
we are counting (p. 77). I will refer to specifications which meet these
criteria as relevant specifications, so that SpecRes is the number
of relevant specifications.
I referred above to the "complexity" of a specification. It is important to
note that this is not the same type of complexity that Dembski defines elsewhere
as a monotonic function of probability (-log2P). According to
Dembski, he is now referring to "a complexity measure φ that characterizes the
complexity of patterns relative to S's [a subject's] background knowledge and
abilities as a cognizer to perceive and generate patterns" (p. 76). The
vagueness of this concept is not helped by a note, in which Dembski gives no
less than three interpretations (p. 118n29):
-
"Computational complexity." This is a measure of the amount of
computational resources (such as computer time and memory) needed to solve a
problem (p. 140).
-
"The degree of compressibility of a specification as measured within
algorithmic information theory." This is Kolmogorov complexity (Dembski
clearly means in-compressibility),41 and
is an attribute of an object, such as a sequence of characters, not an
attribute of a problem, so is not commensurate with computational
complexity.
-
A subject's "disposition to output a given specification." No idea is given
of how such a subjective concept might be measured.
In The Design Inference Dembski referred to this measure as the
computational complexity or degree of difficulty associated with the problem of
formulating a specification. But since he has never told us how specifications
are formulated (i.e. how rejection functions are derived from background
knowledge), this measure is not well-defined. Dembski's claim that "Such a
measure is objectively given (relative to S)" (p. 76) is pure wishful thinking.
I'll refer to this measure as computational complexity.
In the Caputo case, Dembski lists the following potential specifications,
after removing subsets (p. 81):
- All sequences of length 41 with 40 or more Ds.
- All sequences of length 41 with 40 or more Rs.
- All sequences of length 41 consisting of alternating Ds and Rs.
He suggests that there may be additional relevant specifications, but he
thinks the number will not exceed a single digit, and takes the total number as
100 "to play it safe". No attempt is made to calculate the computational
complexity of any specifications. It is just assumed that the computational
complexity of specifications can be compared on some intuitive basis. The first
of the above specifications is the one we chose for calculating
P(R|H), and since the second exhibits an obvious symmetry with the
first, it might be reasonable to take it as having the same computational
complexity, although our background knowledge relating to Democrats is different
from our knowledge relating to Republicans, and one wonders whether that should
have an effect. Since Caputo would be expected to favour his own party, an
outcome matching the first specification would appear more suspicious than one
matching the second. How the computational complexity of the third specification
is compared with that of the other two is a mystery. Finally, we are left to
guess at how many more, unknown relevant specifications there may be. Whether
100 is a conservative overestimate is impossible to judge, given the vagueness
of the criterion by which specifications are compared.
Dembski appears to suggest that the narrower the specification we choose for
calculating P(R|H), the fewer relevant specifications there will
be, and hence the smaller will be SpecRes. This supports the view that
SpecRes compensates for the tailoring of the specification:
By factoring in all the relevant probabilistic resources,
specification effectively precludes unbridled tailoring of events to rejection
regions--each attempt to specify an event incurs a probabilistic cost that has
to be counterbalanced by a probabilistic resource that takes that attempt into
account. [p. 76]
While such a balancing mechanism is clearly welcome, Dembski makes no attempt
to rationalize his particular criteria for calculating SpecRes. My own
interpretation is that he is attempting to count all possible patterns which are
as exceptional, in some sense, as the pattern noted in the observed outcome.
Multiplying P(R|H) by SpecRes can then be interpreted as an
attempt to estimate the probability of a larger rejection region which includes
all the outcomes as exceptional as the observed one. However, given the number
of highly subjective estimates that must be made and the lack of a rational
foundation, it is not clear that this approach offers any advantage over simply
asking the single question: "What proportion of potential outcomes would we
consider as exceptional or more so than the observed one?"
A.3 Replicational Resources
Replicational resources are conceptually much simpler than specificational
resources, but also suffer from the problem of arbitrariness. They are defined
by Dembski as "the number of opportunities for a certain event to occur" (p.
19). For the Caputo case, he provides the following calculation of
ReplRes:
The court is generous in handing out probabilistic resources. The
court imagines that each state in the United States has c = 500 counties (an
exaggeration), that each county has e = 5 elections per year (another
exaggeration), that there were s = 100 states (we imagine rampant American
imperialism doubling the number of states in the union), and that the present
form of government endures y = 500 years (over double the current total). The
product of c times e times s times y equals 125 million and signifies an upper
bound on the total number of elections that might reasonably be expected to
occur throughout U.S. history. These constitute the relevant replicational
resources, which we denote by ReplRes, to account for Caputo giving
Democrats the top ballot line 40 out of 41 times. [p. 81]
Although Dembski has been generous in estimating the values of his parameters
(except, one hopes, for the life expectancy of U.S. democracy), the choice of
parameters is arbitrary. In fact, there was only one opportunity for the
specific observed event to occur. There were, of course, many opportunities for
similar events to occur, but how do we decide how similar an event must
be in order to qualify for inclusion in ReplRes? Why, for example, should
we include county elections but not school board elections? Why elections in the
U.S.A but not elections in other countries? Why not other events which involved
41 trials with just two possible outcomes, such as tossing 41 coins? Dembski's
method forces us to draw an arbitrary line between events which we consider
sufficiently similar to the observed one (such as elections in other states) and
those which we consider insufficiently similar (such as elections in other
countries).
On a point of detail, I note that Dembski counted the number of
individual elections. But the event observed in the Caputo case involved
41 elections supervised by the same clerk. The number of times that one clerk
draws the ballot order for a total of 41 U.S. county elections must be many
times less than the total number of such elections.
From his estimates of SpecRes = 100 and ReplRes = 125 million,
Dembski arrives at a figure of 1 in 25 billion for α, compared to a figure of 1
in 50 billion for P(R|H). He concludes that, since
P(R|H) < α, "The New Jersey Supreme Court is warranted in
inferring that E did not happen in accordance to the chance hypothesis H"
(p. 82). With the inequality decided by a factor of only 2, this conclusion must
be considered highly suspect, given the problems just described.
A.4 The Universal Probability Bound
Dembski recognizes that there is a degree of subjectivity involved in his
method for establishing local probability bounds (at least as far as
SpecRes is concerned), but claims that his universal probability bound
avoids this problem:
Such a universal probability bound takes into account all the
specificational resources that might ever be encountered in the known physical
universe (interestingly, by exhausting the specificational resources of the
universe, we also exhaust all the replicational resources that might ever
arise). [p. 83]
As we saw earlier, the universal probability bound assumes that the maximum
possible number of probabilistic resources is given by:
1080 × 1045 × 1025 =
10150
This is an estimate of the maximum number of elementary particle transitions
that could possibly occur throughout the lifetime of the observable Universe. I
think there are some grounds for questioning whether this actually represents
the maximum possible number of events which could occur, since the same
elementary event can be included in more than one composite event. I will not
pursue this point, however, and will accept that this is the maximum possible
number of replicational resources in the observable universe. It is far less
clear, however, why this figure should be taken as the maximum possible number
of specificational resources, let alone the maximum possible number of combined
probabilistic resources (ReplRes × SpecRes).
Dembski's formal account of specificational resources (p. 77) is based on the
number of potential specifications meeting certain criteria. There is no mention
of any physical constraints on enumerating specifications. There is also no
mention of any such constraint in the Caputo example. But, in order to set a
universal upper limit on SpecRes, Dembski announces that we should only
count the number of physical opportunities to formulate a specification.
The nearest Dembski ever comes to providing a rationale for this constraint
seems to be in the following passages:
That still leaves the problem, however, of a subject identifying
numerous distinct items of background knowledge each of which is conditionally
independent of E given H. Suppose each such item of background
knowledge induces a rejection function that in turn induces a pair of
rejection regions. Then each such rejection region is potentially capable of
eliminating H. Hence by identifying sufficiently many items of such
background knowledge, a subject can in principle run through one rejection
region after another until hitting one that eliminates the chance hypothesis
H. The worry, then, is that the Generic Chance Elimination Argument
might eliminate any chance hypothesis whatsoever. [p. 74]
By factoring in all the relevant probabilistic resources,
specification effectively precludes unbridled tailoring of events to rejection
regions--each attempt to specify an event incurs a probabilistic cost that has
to be counterbalanced by a probabilistic resource that takes that attempt into
account. [p. 76]
Dembski seems to think that the only way the "subject" (the user of his
method) might tailor the specification to the observed outcome is by running
through all conceivable specifications until reaching one which is tightly
tailored to the outcome. But this is a very peculiar conception of how the mind
works. People do not identify patterns by running through every pattern they can
think of and asking whether the observed pattern matches it. This simplistic
idea of human thought processes seems particularly odd coming from Dembski,
given his non-mechanistic view of intelligence (see section 2). Given
the actual ability of people to detect patterns by rapid heuristic methods (as
yet poorly understood), it is irrelevant how many individual specifications a
human mind can elaborate, and Dembski's attempt to set a universal upper limit
to SpecRes is doomed to failure.
Certainly, for many tests, 10-150 can be considered a very
conservative bound. But it cannot be considered a universal bound, and the
judgment of whether it is a suitable bound in a given case will remain a
subjective one.
Incidentally, I could not help but laugh at Dembski's claim that the
subjectivity of his method serves as confirmation of its soundness:
Subjective factors often do influence the setting of probabilistic
resources, and the Generic Chance Elimination Argument faithfully reflects
this fact (further confirmation that this argument schema provides a sound
rational reconstruction of how we eliminate chance). [p. 83]
In fact, all methods of statistical inference involve subjective elements.
The Bayesian and likelihood approaches make this quite explicit. It is, of
course, an advantage to make clear the existence of those subjective elements,
and Dembski does so in this instance but more often fails to do so, as we have
seen. In any case, the idea that the existence of a subjective element indicates
that his particular subjective method is a rational reconstruction of how we
habitually think is absurd in the extreme.
A.5 The "Inflationary Fallacy" Fallacy
Some physicists have proposed that the universe we observe is just one in an
ensemble of many universes. As Dembski points out, there are a number of
different proposals of this sort, including the proposal that the observable
universe is just one region within a vastly larger inflated universe, the
many-worlds interpretation of quantum mechanics, and others. For brevity I will
refer to all such proposals as "multiple universes". Dembski considers the
possible relevance of multiple universes to the estimation of probabilistic
resources, but dismisses the idea that they are relevant as "the inflationary
fallacy" (pp. 86-87). He employs two arguments, neither of which stands up to
scrutiny.
His first argument is that there is no "independent evidence" of multiple
universes (pp. 90-92). Regardless of whether this is true--and I leave that
question to physicists--it does not support Dembski's case. By adopting a purely
eliminative method of inference, he has accepted the burden of rejecting all the
natural hypotheses we can think of. The issue here is not whether the natural
hypotheses have been shown to be true but whether Dembski can show them to be
false. He is now trying to shift the burden of evidence which he voluntarily
took upon himself.
Next, Dembski argues that unlimited probabilistic resources would allow
bizarre possibilities such as Arthur Rubinstein being a world famous pianist yet
knowing nothing at all about music, his music being nothing but an incredibly
fortuitous random pounding on the piano keyboard. In fact, not all multiple
universe hypotheses involve unlimited resources, but let us assume for
the sake of argument that they do. Dembski himself gives the reason why such
bizarre possibilities need not concern us:
Given unlimited probabilistic resources, there is only one way to
rebut this anti-inductive skepticism, and that is to admit that while
unlimited probabilistic resources allow bizarre possibilities like this, these
possibilities are nonetheless highly improbable in the little patch of reality
that we inhabit. Unlimited probabilistic resources make bizarre possibilities
unavoidable on a grand scale. The problem is how to mitigate the craziness
entailed by them, and the only way to do this once such bizarre possibilities
are conceded is to render them improbable on a local scale. Thus in the case
of Arthur Rubinstein, there are worlds where someone named Arthur Rubinstein
is a world famous pianist and does not know the first thing about music. But
it is vastly more probable that in worlds where someone named Arthur
Rubinstein is a world famous pianist, that person is a consummate musician.
What's more, induction tells us that ours is such a world. [p. 93]
Having for once made a cogent argument, but one which counters his own
position, Dembski then spends the next two pages dancing around the issue,
trying to undo his good work, but failing to come up with anything of
substance.
In effect, if multiple universes exist, Dembski's universal probability bound
of 10-150 becomes merely a local probability bound within the
context of the ensemble of universes. For the vast majority of purposes, such as
deciding whether Arthur Rubinstein could have played so well by pure chance, we
need only consider this local probability bound, so the existence of multiple
universes changes nothing. However, when considering the very special case of
the origin of intelligent life, we must take into account the probabilistic
resources of the full ensemble of universes. This is because of the well-known
phenomenon of observational selection effect.59
Even if intelligent life occurs in only an infinitesimal proportion of a vast
ensemble of universes, we should not consider ourselves lucky to find ourselves
in one of those rare universes, since we could not find ourselves in any other.
The same selection effect applies equally to any prerequisite for intelligent
life, such as the origin of life itself. Dembski himself describes and accepts
the relevance of this selection effect with regard to the number of planets on
which life could potentially have originated,60 and
exactly the same argument applies with regard to the number of universes in
which life could potentially have originated.
It must be stressed that my refutation of Dembski's attempt to apply his
design inference to biology does not rely on the existence of multiple
universes. Dembski has failed to establish a small probability for the evolution
of biological structures even relative to his own universal probability bound of
10-150.
A.6 A Rational Foundation?
Howson and Urbach comment as follows on the lack of a rational foundation for
Fisher's approach (this passage is also quoted by Dembski in The Design
Inference):
The force of a test of significance, Fisher then claimed, "is
logically that of the simple disjunction: Either an exceptionally rare
chance has occurred, or the theory of random distribution [i.e., the
null hypothesis] is not true" (Fisher, 1956, p. 39). But in thus avoiding an
unreasonably strong interpretation, Fisher plumped for one that is unhelpfully
weak, for the significant or critical results in a test of significance are by
definition improbable, relative to the null hypothesis. Inevitably, therefore,
the occurrence of a significant result is either a 'rare chance' (an
improbable event) or the null hypothesis is false, or both. And Fisher's claim
amounts to nothing more than this necessary truth. It certainly does not allow
one to infer the truth or falsity of any statistical hypothesis from a
particular result. (Hacking, 1965, p. 81, has made the same point.)
Expositions of Fisherian significance tests typically vacillate
over the nature of the conclusions that such tests entitle one to draw. For
example, Cramér said that when a hypothesis has been rejected by such a
procedure, "we consider the hypothesis is disproved" (1946, p. 334). He
quickly pointed out, though, that "[t]his is, of course, by no means
equivalent to a logical disproof". Cramér contended, however, that
although a rejected theory could in fact be true, when the significance level
is sufficiently small, "we feel practically justified in disregarding
this possibility" (original italics altered). No doubt such feelings do often
arise (though, as we shall see in Chapter 9, section c.3, there are exceptions
to the rule); but Cramér offered no grounds for thinking that such feelings,
when they occur, were generated by the type of reasoning employed in tests of
significance, nor was he able to put those feelings onto any systematic or
rational basis.61
Dembski believes he has accomplished what all other statisticians have failed
to do--put Fisher's approach onto a firm rational foundation. He sums up his
argument in No Free Lunch as follows:
The rationale here is that since factoring in all relevant
probabilistic resources leaves us with an event of probability less than 1/2,
the event is less probable than not, and consequently we should favor the
opposite event, which is more probable than not and precludes it. [p.
79]
The argument is made at greater length in The Design Inference (pp.
193-198), but amounts to no more than this: if the saturated probability
(i.e. the probability after factoring in all the probabilistic resources) of the
event is less than 1/2, we should expect the event not to have occurred; but it
did occur; this is a "probabilistic inconsistency"; to resolve the
inconsistency, we should reject the chance hypothesis which conferred this
probability on the event.
One might think that, if such a simple argument could provide a rational
foundation for Fisher's approach, it would hardly have escaped the notice of the
whole community of statisticians for so long!
In fact, Dembski's conclusion is a non sequitur. He gives no coherent
reason why we should consider the occurrence of an unexpected event to be an
"inconsistency". Unexpected events often do occur, and they do not necessarily
lead us to revise the beliefs which rendered them unexpected.
Since Dembski is making a very ambitious claim, the onus is on him to make a
sound argument in support of it, and he has signally failed to do so.
Nevertheless, though not required to, I will give some counterexamples.
Consider this example: I toss a coin twice as a test of its fairness,
specifying in advance that I will reject the hypothesis of fairness (that each
toss has a 1/2 probability of resulting in a head) if I obtain two heads. To
ensure that there will be only one trial of that coin, a newly minted coin is
selected and the coin is destroyed after the trial. Since there can only be one
trial, the number of replicational resources is 1. Since I have provided the
specification in advance, there is only one possible specification and so the
number of specificational resources is 1. Therefore the saturated probability of
the event is 1/4. If I then proceed to obtain two heads, I should, apparently,
infer that the coin was unfair. But I doubt that any reader of this article
would draw that inference.
A similar example appears in The Design Inference (pp. 196-197), where
Dembski insists that the subject must count as replicational resources all the
coin tosses that he has made throughout his life. In fact, unlike my example,
Dembski's subject did not stipulate in advance that the trial would be
considered a test of the fairness of the coin or that there would only be one
trial, so the examples are not equivalent. In any case, we could imagine, for
the sake of argument, that I am replaced in my example with a person who has
never tossed a coin before and swears never to do so again. Nevertheless,
although I can see no grounds for him to do so, I suspect that Dembski will try
to evade the force of this counterexample by claiming that we must count all the
coin tosses ever made (or likely to be made) throughout the course of human
existence. In case he should do so, I offer the following additional
examples:
-
Consider a thought experiment in which a trial of the sort described above
is the only time that coins are tossed throughout the whole existence of the
human race.
-
Imagine the first trial of some radically new technological process, which
sadly results in the destruction of the world. The only survivors are the
inhabitants of a small lunar colony, who do not have the technology to repeat
the trial and will not survive for long enough to obtain it. As it happens,
one of the colonists had an interest in the new technology, and before the
trial, specified the trial as a test of his hypothesis that the probability of
global destruction was no more than 1/3. After the destruction, should he
reject this hypothesis?
-
Consider the argument from cosmological fine-tuning, which attempts to
infer design from the alleged minuscule probability of obtaining a
life-enabling universe by random generation of the cosmological constants. If
we were to accept Dembski's conclusion, then proponents of this argument would
only need to show that the probability of a life-enabling universe was less
than 1/2, rather than the vastly smaller probability which is actually
claimed. The number of replicational resources in this case is only 1, since
the argument is based on the assumption that there is only one universe. The
number of specificational resources is also 1, given the specification
"life-enabling universe", since we could not possibly have observed any other
outcome. I doubt that the cosmological fine-tuning argument would enjoy its
current popularity (or indeed any popularity at all) if the probability of a
life-enabling universe had been shown to be 1/3.
A.7 Conclusion of the Appendix
Dembski's claim to have provided a rational foundation for Fisher's method of
hypothesis testing is quite untrue, and his own version of the method suffers
from much the same problems of arbitrariness as Fisher's. But does Dembski's
method offer any advantages at all over Fisher's? It might be argued that, by
trying to count the number of probabilistic resources, however subjectively,
Dembski has at least broken down one subjective judgment (regarding the size of
the probability bound) into a number of smaller, more manageable ones. Perhaps.
But any such minor advantage must be offset against the fact that Dembski has
allowed the user of the method much greater freedom to tailor the rejection
region to the observed outcome, and against the confusion caused by Dembski's
convoluted and equivocal exposition of what is really rather a trivial
idea.
Notes
1. William Dembski, No Free Lunch: Why Specified
Complexity Cannot be Purchased without Intelligence, Rowman &
Littlefield, 2002.
2. I do not attempt to draw a hard line of demarcation
between science and pseudoscience. By pseudoscience I mean egregiously bad
science. See also: "pseudoscience", The Skeptic's Dictionary.
3. Richard Wein, "What's Wrong With The Design Inference",
Metaviews online forum, October 2000.
4. William Dembski, "The Design Inference: Eliminating Chance
Through Small Probabilities", Cambridge University Press, 1998.
5. William Dembski, "Intelligent Design Coming Clean",
Metaviews online forum, November 2000.
6. My own view is that methodological naturalism is an
ill-defined and arbitrary restriction which should be abandoned. The principle
of "extraordinary claims require extraordinary evidence" is sufficient to
prevent premature appeals to unembodied beings, but there is no reason to rule
such beings out of science for all time regardless of what evidence might
appear.
7. An outcome is an event which cannot be
subdivided. It is also known as an elementary event. For example, in the
Caputo case, each possible sequence of 41 Ds and Rs is considered a different
outcome. The set of all such outcomes is known as the outcome space or
sample space. (Dembski uses the term phase space.) Events such as
"40 or more Ds" and "the 5th draw is a D" are known as composite events,
because they consist of more than one outcome.
8. William Dembski, "The Explanatory Filter: A three-part
filter for understanding how to separate and identify cause from intelligent
design", 1996.
9. The term local probability bound occurs in
Dembski's earlier work and not in No Free Lunch, but I find it convenient
to use it here.
10. Dembski is rather vague about what inference should be
drawn in the Caputo case. He considers only one chance hypothesis, namely the
fair draw hypothesis H. Having rejected this hypothesis, Dembski does not
clearly state that we should infer design, merely writing:
Step #8 [of the Generic Chance Elimination Argument] now follows
immediately: The New Jersey Supreme Court is warranted in inferring that E did
not happen according to the chance hypothesis H. [p. 82]
In stating that "Step #8 now follows immediately", he implies that we should
infer specified complexity, and presumably an inference of design follows. But,
as this is Dembski's only complete example of a design inference (other than the
highly questionable case of the bacterial flagellum), a clear statement of the
result might have been expected.
How sure are we that no other natural causes could have been operating?
Dembski argues that H is "the only chance hypothesis that could have been
operating to produce E... because Caputo himself was responsible for the ballot
selections and claims to have used this chance process" (p. 80). He rules out
the possibility that Caputo's randomization procedure was innocently flawed, on
the grounds that Caputo drew capsules from a container, and that "urn models are
among the most reliable randomization techniques available" (p. 56). Presumably
this rejection of the possibility of a flawed urn procedure should be considered
a proscriptive generalization. But what if Caputo was lying about the process he
used, and actually used some other process which he thought to be fair but was
not? It seems Dembski would still count this as "design".
So it appears that the inference of design in this case represents several
possibilities: Caputo cheated, Caputo lied about his randomization method, or
some other intelligent agent (perhaps unembodied) interfered in the process.
11. "Occam's Razor", Principia Cybernetica Web.
12. Dembski frequently claims that a certain event or
phenomenon exhibits specified complexity, without having performed an explicit
probability calculation. I take the liberty here of doing the same.
13. William Dembski, "The Intelligent Design Movement",
Cosmic Pursuit, Spring 1998.
14. In support of their argument from the probability of
purely random combination, Creationists often quote the astronomer Fred Hoyle:
"The current scenario of the origin of life is about as likely as a tornado
passing through a junkyard beside Boeing airplane company accidentally producing
a 747 airplane."
15. There are many web pages rebutting Behe's arguments in
detail, including the following: - Kenneth Miller, "Design on the Defensive",
. -
Don Lindsay, "Review: 'Darwin's Black Box, The Biochemical Challenge to
Evolution' by Michael J. Behe", August 2000. -
"Irreducible Complexity and Michael Behe", The Talk.Origins Archive.
16. Michael Behe, Darwin's Black Box (Simon &
Schuster, 1998), pp. 39-40.
17. Michael Behe, "Self-Organization and Irreducibly
Complex Systems: A Reply to Shanks and Joplin", Philosophy of Science 67
(1), 2000:
Systems requiring several parts to function that need not be
well-matched, we can call "simple interactive" systems (designated 'SI'). Ones
that require well-matched components are irreducibly complex ('IC'). The line
dividing SI and IC systems is not sharp, because assignment to one or the
other category is based on probabilistic factors which often are hard to
calculate and generally have to be intuitively estimated based on
always-incomplete background knowledge. Moreover, no law of physics
automatically rules out the chance origin of even the most intricate IC
system. As complexity increases, however, the odds become so abysmally low
that we reject chance as an explanation (Dembski 1998).
18. Kenneth Miller, "The Evolution of Vertebrate Blood
Clotting". -
Don Lindsay, "How Could The Immune System Evolve?", April 1999. -
Mike Coon, "Is the Complement System Irreducibly Complex?", The Talk.Origins
Archive, February 2002. -
Ian Musgrave, "Evolution of the Bacterial Flagella", March 2000.
An updated version of this web page is currently in preparation.
19. Michael Behe, "Reply to My Critics: A Response to
Reviews of Darwin's Black Box: The Biochemical Challenge to Evolution",
Biology and Philosophy 16: 685–709, 2001:
It is now clear that, although the mousetrap paradigm remains a
good one, there is some ambiguity in the written definition, as discussed
below. Nonetheless, I think the definition can be repaired.
20. Michael Behe, "A Response to Critics of Darwin's
Black Box", Discovery Institute, December 2001:
Envisioning IC in terms of selected or unselected steps thus puts the focus
on the process of trying to build the system. A big advantage, I think, is
that it encourages people to pay attention to details; hopefully it would
encourage really detailed scenarios by proponents of Darwinism (ones that
might be checked experimentally) and discourage just-so stories that leap over
many steps without comment. So with those thoughts in mind, I offer the
following tentative evolutionary definition of irreducible complexity:
An irreducibly complex evolutionary pathway is one that contains
one or more unselected steps (that is, one or more necessary-but-unselected
mutations). The degree of irreducible complexity is the number of unselected
steps in the pathway.
21. I use the term functional complexity in a loose
sense to refer to the sort of complexity which we intuitively recognize when we
look at machines and organisms.
22. Dembski quotes Joseph Culberson in a passage which
appears to use the term blind search in Dembski's second sense (p. 196).
But careful reading of Culberson reveals that he is using the term to mean
any black-box algorithm: "The environment acts as a black box, and so we
refer to this as the black box or blind search model.... [Wolpert
and Macready] prove within a formal setting that all optimization
algorithms have equivalent average behavior when pitted against such a black box
environment." Joseph Culberson, "On the Futility of Blind Search",
Evolutionary Computation 6(2), 1998. An earlier version of the paper can
be found online.
23. William Dembski, "Why Natural Selection Can't Design
Anything", 2001.
24. Geoffrey Miller, "Technological Evolution As
Self-Fulfilling Prophecy", in J. Ziman (Ed.), Technological innovation as an
evolutionary process (Cambridge U. Press, 2000). pp. 203-215.
25. David Wolpert and William Macready, "No Free Lunch
Theorems for Search", Santa Fe Institute Technical Report 95-02-010, 1995.
26. Since Richard Dawkins' Weasel program will already be
familiar to many readers, I will not describe it here. Dawkins' original
description can be found in The Blind Watchmaker (Penguin, 1991), pp.
45-50. A brief account can be found online at The Evolution of Improved Fitness
By Random Mutation Plus Selection,
section 1.2.3. Dembski's description of the program in No Free Lunch
contains serious errors.
Dembski also follows in a long line of Creationists and Intelligent Design
proponents who have criticized the Weasel program for failing to be something
which Dawkins has never claimed it to be. According to Dembski, the program is
purported by Dawkins "to show how an evolutionary algorithm can generate
specified complexity" (p. 181). In reality, Dawkins neither calls the Weasel
program an evolutionary algorithm nor claims that it can "generate" complexity
of any sort. The sole purpose of the Weasel program was to illustrate the
difference between single-step selection and cumulative selection.
(These two terms are described in 6.8 above.) Dawkins
is very careful to state this clearly, though apparently not clearly enough for
antievolutionists. Dembski quotes the following passage from Dawkins, but
appears not to have understood it:
Although the monkey/Shakespeare model is useful for explaining the
distinction between single-step selection and cumulative selection, it is
misleading in important ways. One of these is that, in each generation of
selective "breeding", the mutant "progeny" phrases were judged according to
the criterion of resemblance to a distant ideal target, the phrase
METHINKS IT IS LIKE A WEASEL. Life isn't like that. Evolution has no long-term
goal. [p. 208]
Dembski also presents a revised version of the program, and then makes the
absurd claim that his version, unlike Dawkins' original, does not involve an
element of "teleology" because it searches the phase space "without
explicit recourse to the target" (p. 193). In reality both versions
described by Dembski make explicit recourse to the target, comparing each trial
sequence against the target sequence.
27. See, for example, Hugh Ross, "Design and the Anthropic
Principle". For
a refutation of the cosmological fine-tuning argument, see Theodore Drange, "The
Fine-Tuning Argument Revisited (2000)", Philo 2000 (Vol. 3, No. 2).
28. David Wolpert and William Macready, "No Free Lunch
Theorems for Optimization", IEEE Transactions on Evolutionary
Computation, 1(1):67-82, April 1997.
29. In terms of a computer program, we can think of the
fitness function being updated by another module, separate from the optimization
algorithm. Because of the black-box constraint, this additional module,
representing external factors, is not allowed to communicate with the algorithm
module in any other way if we want NFL to apply.
30. Thomas Jansen, "On Classifications of Fitness
Functions", 1999.
See also: - Stefan Droste, Thomas Jansen and Ingo Wegener, "Perhaps Not a
Free Lunch But At Least a Free Appetizer", Proceedings of the 1st Genetic and
Evolutionary Computation Conference (July 13-17, 1999, Orlando, FL), pages
833-839. -
Oliver Sharpe, "Beyond NFL: A Few Tentative Steps", Genetic Programming 1998:
Proceedings of the Third Annual Conference (July 22-25, 1998, University of
Wisconsin). -
Christian Igel and Marc Toussaint, "On Classes of Functions for which No Free
Lunch Results Hold", 2001, submitted to IEEE Transactions on Evolutionary
Computation
31. Dembski establishes a higher-order fitness function F
on the phase space Ω × J, where Ω is the original phase
space and J is the set of all possible fitness functions on Ω. He
then considers a search over this space, i.e. a sequence of ordered pairs
(xi, fi), where xi is in Ω and
fi is in J, with Fi = fi(xi).
Dembski's F is equivalent to the time-dependent fitness function T considered by
Wolpert and Macready.28 In
Wolpert and Macready's NFL Theorem 2, T is independent of the algorithm, so it
cannot take coevolution into account. With coevolution, fi is
dependent on the current state of the population.
32. Dembski is inconsistent in his usage of the terms
specified information and CSI. Sometimes they are "items" of the form (T,
E), where E is an observed outcome and T (or target) is another name for
a detachable rejection region R (pp. 142-143). Sometimes they are properties
which are either exhibited or not by a phenomenon (p. 151). Sometimes they are
quantities: "Because small amounts of specified information can be produced by
chance..." (p. 161); "The CSI in a closed system of natural causes remains
constant or decreases" (p. 163). To avoid long-winded expressions such as "the
quantity of information in an item of specified information" (p. 160), I will
use specified information (or SI) in the last of these three senses, i.e. as a
quantity. I will use CSI in the second sense, i.e. as an attribute which is
either exhibited or not. It should be understood that the probability used in
calculating SI or CSI is always the probability of a detachable rejection region
R, and not just the probability of the observed outcome E on its own.
33. Thomas Schneider, "Evolution of Biological
Information", Nucleic Acids Research, 28(14): 2794-2799, 2000.
Also at this site you can find Schneider's response to Dembski's treatment of
his work: "Rebuttal to William A. Dembski's Posting and to His Book 'No Free
Lunch'", March 9 2002;
and a useful "Information Theory Primer".
34. Perhaps Dembski's choice of terminology has
helped confuse him. The phase space of an optimization algorithm is normally
called a search space, while the phase space of a probability
distribution is called an outcome space or sample space. By always
using the term phase space regardless of the context, Dembski has blurred
this distinction.
35. It isn't clear whether Dembski considers this to be
38 bits of specified complexity/information (SI). He does say that the
sequence METHINKS is specified, insofar as it is a "known word in the English
language". But, if the specification is "known word in the English language", he
needs to calculate the probability of drawing any English word of 8
letters, giving a rather lower complexity value. In fact, Dembski only writes
that the complexity is bounded by 38 bits, not that it is
precisely 38 bits. Perhaps this was to allow for alternative words of 8
letters.
36. I have found in past discussions of Dembski's work
that considerable confusion has been caused by his misuse of Shannon information
theory. Although not essential to my critique, I will attempt here to clear up
some of this confusion. My main source is The Mathematical Theory of
Communication (Univ. of Illinois Press, 1949). This small book consists of
two papers, one each by Claude Shannon and Warren Weaver. An earlier (1948) but
largely identical version of Shannon's paper is available online.
For a more gentle online introduction to information theory, see "Information Theory Primer".
Shannon information theory is concerned with the transmission of messages
through a communications channel. The meaning of the messages is
immaterial. All that matters is the efficiency and accuracy with which messages
are transmitted. Messages are treated as if they were selected at random from an
ensemble of possible messages. This means that the same theory can also be used
in relation to other types of probabilistic events, in which the occurrence of
one outcome is observed out of a set of possible outcomes.
The rate of transmission of information is defined by Shannon as follows:
R = H(x) - Hy(x)
If we think in terms of transmitting a message, drawn randomly from a set of
possible messages, from a transmitter to a receiver, then H(x) is the receiver's
uncertainty about which message was (or will be) transmitted
before receiving any message. Hy(x) is the receiver's
uncertainty about which message was transmitted after receiving a
message. R can also be thought of as the reduction in uncertainty as a result of
receiving the message. If the channel is free of noise--so the message received
is always the same one that was sent--then Hy(x) = 0 and R =
H(x).
The uncertainty H(x) (or simply H) is defined as follows:
H = - Σi=1...N pi
log2pi
where there are N possible messages and the probability of message i
being transmitted is pi.
It is important to bear in mind that R and H are rates, or averages (weighted
by probability), based on the ensemble of all messages which could possibly be
transmitted. They are not values associated with the receipt of one particular
message. There is, however, another measure, defined as
-log2pi, which is associated with receipt of
a specific message. It is sometimes known as surprisal, after M. Tribus
[Thermostatics and Thermodynamics (D. van Nostrand Co., 1961)], as it
indicates how surprised we should be at receiving that message. The uncertainty
H is then equal to the average surprisal, over all possible messages, weighted
by probability.
There appears to be some disagreement about which measure is correctly known
as the Shannon information. Some writers, including Dembski, refer to the
surprisal as the Shannon information associated with the receipt of one specific
message. The thinking seems to be that, if H is the rate of transmission
of information averaged over all possible messages, then the surprisal must be
the information associated with the receipt of one particular message.
However, this is not the usage of Shannon or Weaver, who refer to R as the
information. Consequently, most information theorists refer to R as the Shannon
information. The expression -log2pi appears nowhere
in the Shannon and Weaver papers. For a noise-free channel, Shannon equates
information with uncertainty:
The quantity H has a number of interesting properties which
further substantiate it as a reasonable measure of choice or information.
[Shannon & Weaver, 1949, p. 51]
Weaver is more explicit, and makes it clear that information is a property of
the ensemble of possible messages, not of one particular message:
To be sure, this word information in communication theory relates
not so much to what you do say, as to what you could say. That
is, information is a measure of one's freedom of choice when one selects a
message. If one is confronted with a very elementary situation where he has to
choose one of two alternative messages, then it is arbitrarily said that the
information, associated with this situation, is unity. Note that it is
misleading (although often convenient) to say that one or the other message
conveys unit information. The concept of information applies not to the
individual messages (as the concept of meaning would), but rather to the
situation as a whole, the unit information indicating that in this situation
one has an amount of freedom of choice, in selecting a message, which it is
convenient to regard as a standard or unit amount. [Shannon & Weaver,
1949, pp. 8-9]
Note that, when all the possible outcomes are equally probable (i.e.
pi is a constant p) the uncertainty reduces to -
log2p:
H = - Σi=1...N pi
log2pi = - N .
p log2p = - N . 1/N .
log2p = -
log2p
This must not be confused with the surprisal, although it has the same
formula. For an ensemble of possible outcomes which are all equally probable,
the surprisal of each outcome just happens to equal the uncertainty of the
ensemble.
For the sake of an example, consider a 5-card hand dealt from a well-shuffled
deck of 52 cards. There are (52×51×50×49×48)/(5×4×3×2×1) = about 2 million
possible outcomes. Since all outcomes are equally probable p = 0.0000005,
and the uncertainty (H) associated with the deal is -log2(0.0000005)
= 21 bits, using the special formula for equiprobable distributions just derived
above. Once we have seen the 5 cards there is no uncertainty about what was
dealt, so Hy(x) = 0, and the Shannon information is given by R = 21 -
0 = 21 bits.
Now suppose that, as in Dembski's example (pp. 126-127), a royal flush
(10-J-Q-K-A in one suit) is dealt. There are 4 possible royal flushes (one in
each suit), so the probability of a royal flush of any suit is 4 × 0.0000005 =
0.000002. The surprisal of this event is therefore -log2(0.000002) =
19 bits.
Like some other writers, Dembski refers to the surprisal
(-log2pi) as Shannon information (p. 230n16). This
in itself is not particularly important. What matters is not what he calls this
measure but how he uses it. The trouble is that he merely uses it as a disguised
probability measure. The function f(x) = -log2x is a
monotonic function, which means that greater surprisal always corresponds to
greater improbability. Every one of Dembski's statements about information could
just as well (and with much greater clarity) be expressed as a statement about
improbability. He often uses the terms improbability, information and complexity
interchangeably. In the index of The Design Inference he even has an
entry for "probability... information in disguise". By disguising his
probabilities as information, Dembski simply adds another layer of obfuscation
to his arguments, without achieving anything of value.
37. Kumar Chellapilla and David Fogel, "Co-Evolving
Checkers Playing Programs using only Win, Lose, or Draw", SPIE's
AeroSense'99: Applications and Science of Computational Intelligence II
(Apr. 5-9, 1999, Orlando, Florida).
38. Strictly speaking, each neural net in a generation has a
different fitness function, since its environment (the population of other
neural nets) is different.
39. This is pessimistic. A modest overlap of ranges (e.g.
W- a little smaller than D+) may still give a good
result.
40. Chellapilla and Fogel's scoring regime is the one in
which W- = W+ = +1, D- = D+ = 0, and
L- = L+ = -2.
41. The algorithmic information or Kolmogorov
complexity of a sequence is the length of the shortest program which is able
to generate the sequence. It is therefore a measure of incompressibility.
42. Paul Davies, The Fifth Miracle (The Penguin
Press, 1998), pp. 85-89.
43. Leslie Orgel, The Origins of Life (Chapman and
Hall, 1973), p. 190.
44. Dawkins, on the other hand, does adopt a definition
of complexity based on probability under a uniform probability distribution, and
perhaps this is where Dembski obtained the idea. In The Blind Watchmaker
Dawkins writes:
Let us try another tack in our quest for a definition of
complexity, and make use of the mathematical idea of probability. Suppose we
try out the following definition: a complex thing is something whose
constituent parts are arranged in a way that is unlikely to have arisen by
chance alone. To borrow an analogy from an eminent astronomer, if you take the
parts of an airliner and jumble them up at random, the likelihood that you
would happen to assemble a working Boeing is vanishingly small. There are
billions of possible ways of putting together the bits of an airliner, and
only one, or very few, of them would actually be an airliner. [Richard
Dawkins, The Blind Watchmaker (Penguin, 1991), p. 7.]
Although Dawkins also employs the term specified, it is not clear that
his term means the same as Dembski's. Although he is not quite explicit, it
seems that Dawkins requires us to consider all functions that the object
might have had, and not just the particular function that we observe, as
Dembski's concept allows. Furthermore, Dawkins briefly mentions an additional
criterion of heterogeneity, which perhaps saves highly patterned
phenomena from being classified as complex, as happens with Dembski's version.
Dawkins' definition of complexity may be flawed too, but this is relatively
unimportant, since he uses it only to clarify what sort of systems he is talking
about, and it does not play a significant part in his argument:
I am aware that my characterization of a complex
object--statistically improbable in a direction that is specified not with
hindsight--may seem idiosyncratic.... If you prefer some other way of defining
complexity, I don't care and I would be happy to go along with your definition
for the sake of discussion. [Richard Dawkins, The Blind Watchmaker, p.
15.]
45. Richard Dawkins, The Blind Watchmaker, pp.
45-50.
46. The complete list can be found at: William Dembski,
"Intelligent Design Coming Clean", Metaviews online forum, November 2000.
47. William Dembski, "Is Intelligent Design Testable?",
Metaviews online forum, January 2001.
48. Douglas Theobald, "The Opportunistic Nature of Evolution
and Evolutionary Constraint", The Talk.Origins Archive -
Chris Colby & Loren Petrich, "Evidence for Jury-Rigged Design in Nature",
The Talk.Origins Archive.
49. For a list of predictions of evolutionary theory,
see: - Douglas Theobald, "29 Evidences for Macroevolution", The Talk.Origins
Archive, March 2002. -
Don Lindsay, "Is Evolution Science?", August 2001.
50. Douglas Theobald, "The One True Phylogenetic Tree", The
Talk.Origins Archive. It
will do Dembski no good to point out that there are a few exceptions to this
congruence. The methods for establishing phylogenetic trees are fallible. The
prediction is only that there will be a high degree of congruence, not perfect
congruence.
51. Beth McMurtrie, "Darwinism Under Attack", The
Chronicle of Higher Education, December 21, 2001.
52. The following endorsement appeared on the cover of
Dembski's book Intelligent Design: The Bridge Between Science &
Theology:
William Dembski is the Isaac Newton of information theory, and
since this is the Age of Information, that makes Dembski one of the most
important thinkers of our time. His "law of conservation of information"
represents a revolutionary breakthrough. In Intelligent Design: The Bridge
Between Science & Theology, Dembski explains the meaning and significance
of his discoveries with such clarity that the general public can readily grasp
them. He convincingly diagnoses our present confusions about the relationship
between science and theology and offers a promising alternative. -- Rob
Koons, Associate Professor of Philosophy, University of Texas at
Austin
Dembski and Koons are both fellows of the Discovery Institute's Center for
the Renewal of Science & Culture, a
body which exists specifically for the purpose of promoting Intelligent Design.
This sort of mutual puffing of each other's books is commonplace among fellows
of the Center.
53. Keith Devlin, "Snake Eyes in the Garden of Eden",
The Sciences, July/August 2000.
54. Keith Devlin concurs with this assessment. (Personal
communication.)
55. In response to my enquiry on the subject, Sam
Northshield (the author of the notice) replied: "The work that I reviewed was,
as I remember, definitely more philosophical than mathematical and I judged it
as a philosophical work. I don't remember trying hard to understand its math
content (either because it seemed difficult to follow or because there was
none-- I don't remember which!). Therefore, I can't say anything about the
correctness of Dembski's work and my review should not be construed as a
mathematical judgement of the work." (Personal communication.)
56. Colin Howson & Peter Urbach, Scientific
Reasoning: The Bayesian Approach (Open Court, 1993).
57. ibid., p. 181.
58. Theodore Drange, "The Fine-Tuning Argument (1998)".
59. For a very detailed analysis of observational
selection effects, see Nick Bostrom, "Observational Selection Effects and
Probability", 2000.
60. Dembski, The Design Inference, pp. 182-183.
61. Howson & Urbach, Scientific Reasoning,
pp. 179-180 (quoted by Dembski, The Design Inference, pp. 199-200).
This article first appeared in the Talk.Origins Archive.
|
 |